Abstract
Extraction of low-dimensional latent space from high-dimensional observation data is essential to construct a real-time robot controller with a world model on the extracted latent space. However, there is no established method for tuning the dimension size of the latent space automatically, suffering from finding the necessary and sufficient dimension size, i.e. the minimal realization of the world model. In this study, we analyze and improve Tsallis-based variational autoencoder (q-VAE), and reveal that, under an appropriate configuration, it always facilitates making the latent space sparse. Even if the dimension size of the pre-specified latent space is redundant compared to the minimal realization, this sparsification collapses unnecessary dimensions, allowing for easy removal of them. We experimentally verified the benefits of the sparsification by the proposed method that it can easily find the necessary and sufficient six dimensions for a reaching task with a mobile manipulator that requires a six-dimensional state space. Moreover, by planning with such a minimal-realization world model learned in the extracted dimensions, the proposed method was able to exert a more optimal action sequence in real-time, reducing the reaching accomplishment time by around 20%.
GRAPHICAL ABSTRACT
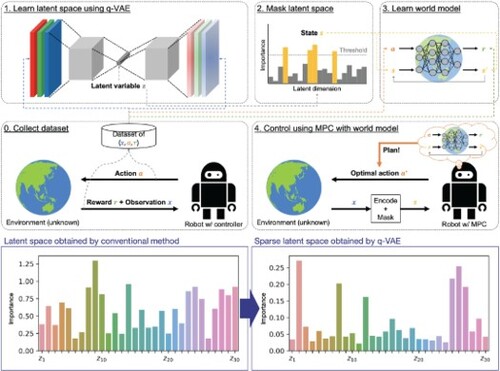
Disclosure statement
No potential conflict of interest was reported by the author(s).
Additional information
Funding
Notes on contributors
Taisuke Kobayashi
Taisuke Kobayashi received his B.E., M.E., and Ph.D. degrees from Nagoya University, Aichi, Japan, in 2012, 2014, and 2016, respectively. From 2018 to 2019, he was a visiting scholar of the Technical University of Munich, Munich, Germany. From 2016 to 2022, He was an Assistant Professor of the Nara Institute of Science and Technology, Nara, Japan. Since 2022, he is an Assistant Professor of the National Institute of Informatics, Tokyo, Japan. His research interests include the robot motion control with machine learning.
Ryoma Watanuki
Ryoma Watanuki received his B.E. in National Institute of Technology, Matsue College, Shimane, Japan, in 2020 and his M.E. in Nara Institute of Science and Technology, Nara, Japan, in 2022. His research interest is in variational autoencoder.