ABSTRACT
In this paper, a new method based on the Tufts–Kumaresan (TK) algorithm is used to process nonstationary signals and extract system modes. This new algorithm for mode estimation is proposed to improve estimation accuracy under dynamic conditions. The key contribution in TK is the use of multiple orthogonal sliding windows rather than a single pair of sliding windows. Simulation results under various scenarios, such as different types of faults and load changes are used to evaluate the performance of the proposed method. The proposed method will accurately extract system modes. This method will also reduce the required memory and the calculation time of estimation in nonstationary signals. In complement to successful applications in studying the dynamic behavior of the power system, identifying and analyzing low-frequency electromechanical oscillations, and removing signal noise, this method can accurately estimate the modes of a power system. To validate the accuracy of the proposed method, the Wavelet and the Prony methods have been used for comparison. The proposed method is implemented using simulated ringdown data of standard two-area power system and real measurement data of the WSCC system breakup on 10 August 1996.
Disclosure statement
No potential conflict of interest was reported by the author(s).
Notes
1. Error Feedback Lattice Recursive Least Squares
2. Recursive Least Squares
3. Discrete Taylor Fourier Transform
4. Stochastic Subspace Identification
5. Variation Mode Decomposition
6. Transient Index Value
Additional information
Notes on contributors

Majid Alizadeh Moghdam
Majid Alizadeh was born in Iran in 1988. He received the MSc degree in electrical engineering from Zanjan University, Zanjan, Iran, in 2014. He is currently a PhD student at the University of Zanjan. His research interests include power system dynamic, renewable energy, distributed generation, power system operation, power electronics, and artificial intelligence.
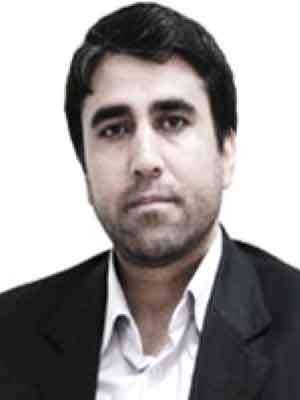
Reza Noroozian
Reza Noroozian (M’09) was born in Iran. He received the BSc degree in power systems from Tabriz University, Tabriz, Iran, in 2000, and the MSc and PhD degrees in electrical engineering
from Amirkabir University of Technology, Tehran, Iran, in 2003 and 2008, respectively. He is an associate professor with the Department of Power Engineering, The University of Zanjan, Zanjan, Iran. His areas of interest include power electronics, power systems, power quality, integration and control of renewable generation units, custom power, micro-grid operation, distributed-generation modeling, as well as operation and interface control.
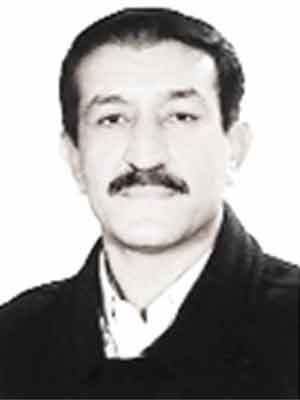
Saeed Jalilzadeh
Saeed Jalilzadeh was born in Iran. He received the BSc and MSc degrees in power systems from Tabriz University, Tabriz, Iran, in 1987 and 1991, respectively, and the PhD degree in electrical engineering Iran University of Science and Technology, Tehran, Iran, in 2005. He is an associate professor with the Department of Power Engineering, The University of Zanjan, Zanjan, Iran. His areas of interest include power system operation, power systems, power quality, integration and control of renewable generation units, custom power.