Figures & data
Figure 2. Illustration of the smoothing method performance on two asset (1,2) portfolio selection. Examples of the trajectories of the method for different discontinuous penalties. ,
.
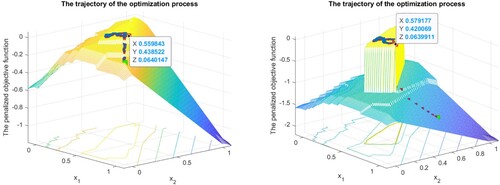
Figure 3. Illustration of the smoothing method performance on two asset (4,9) portfolio selection. Example of the trajectory of the method for non-connected feasible set. ,
. The green is the starting point, the red is the final one.
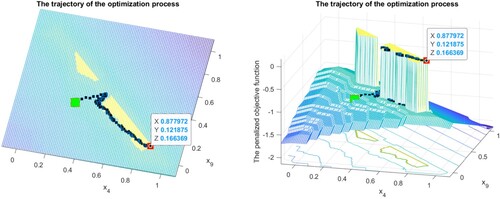
Figure 4. Profiles of optimal 3 component portfolios: maximizing the tail returns under the risk-return lower bound. Discontinuous penalties. 1) Optimal average return. 2) Optimal . 3) Optimal
; cf. Table .
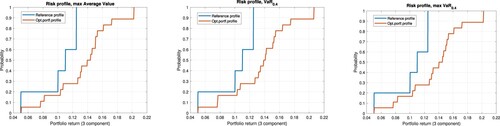
Figure 5. Profiles of optimal 3 component portfolios: maximizing the tail returns under the risk-return lower bound. Analytical projective penalties. (1) Optimal average return. (2) Optimal . (3) Optimal
. See Table .
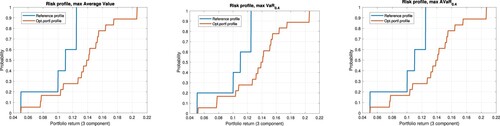
Table 1. Optimal 3 component portfolios, discontinuous penalties: (1) Max mean; (2) Max ; (3) Max
.
Table 2. Optimal 3 component portfolios, analytical projection: (1) Max mean. (2) Max . (3) Max
.
Figure 6. Profiles of optimal 3 component portfolios: maximizing the tail returns under the risk-return lower bound. Analytical projective penalties. (1) Optimal average return. (2) Optimal . (3) Optimal
. See Table .
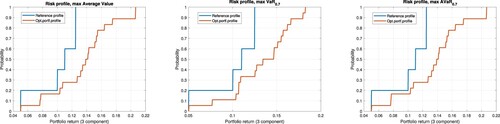
Figure 7. Profiles of optimal 10 component portfolios: maximizing the tail returns under the risk-return lower bound. Analytical projective penalties. (1) optimal average return; (2) optimal ; (3) optimal
; cf. Tables and .
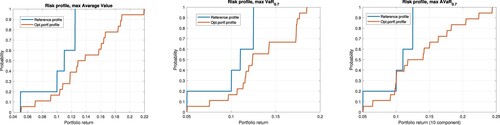
Table 3. Optimal 3 component portfolios, analytical projection: (1) Max mean. (2) Max . (3) Max
.
Table 4. Optimal 10 component portfolios, analytical projection: (1) Max mean. (2) Max . (3) Max
.
Table 5. The optimal 10 component portfolio.
Table A1. Return data set from [Citation62, Table 1, page 13], with artificial bond column.