Figures & data
Table 1 Summary of records used in this study. “Start” and “end” are the first and last years of the time series. Acronyms are as follows. NOAA: US National Oceanic and Atmospheric Administration; JISAO: Joint Institute for the Study of the Atmosphere and Ocean; AHCCD: Adjusted Historical Canadian Climate Data; WSC: Water Survey of Canada; RHBN: Reference Hydrometric Basin Network; INSTAAR: Institute of Arctic and Alpine Research, University of Colorado at Boulder.
Fig. 1 Illustrative examples of numerical power spectra. Thin, solid, dark grey line gives best-fit regression between ln(f) vs ln(PSD); thick, dashed, light grey vertical line is ln(f *). (a) PDO index (circulation pattern, outcome 2). (b) Central England mean temperature (climate station, outcome 3). (c) Bow River maximum flow (water resources, outcome 1a) (f * undefined and not shown; see Table 2). (d) Storglaciären net balance (glacier mass balance, outcome 2).
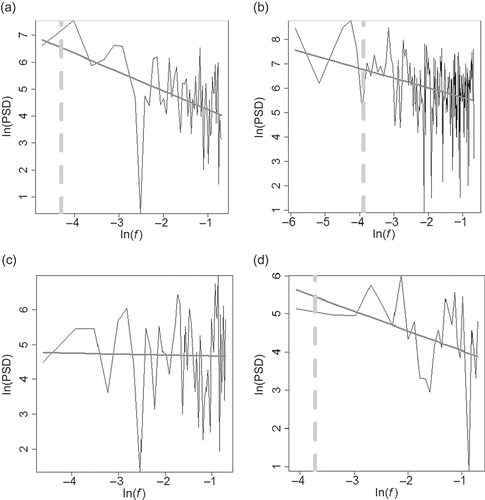
Table 2 Analysis results. “Log regression” gives regression slope, β1, and significance of correlation, p, corresponding to potential linear associations between ln(f) vs ln(PSD) for each time series. “Heuristic rule” gives data set duration, T; observed serial correlation coefficient of the observational time series, ρ; critical timescale from heuristic rule, τ*; natural logarithm of Rayleigh frequency corresponding to T, ln(fRay); and logarithm of frequency corresponding to τ*, ln(f *). “Outcome” summarizes the assessment of background spectrum form for each time series, using both quantitative information and visual spectrum assessments; the numbers correspond to the numbered outcomes discussed in the text.