Figures & data
Figure 2. Plots of autocorrelation: (a) discharge; and cross-correlation of (b) discharge–rainfall and (c) discharge–evapotranspiration.
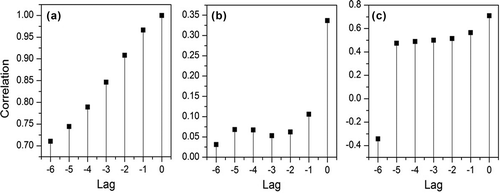
Table 1. Statistical properties of the ANN parameters optimized for Case I and Case II. SD: standard deviation.
Table 2. Summary statistics and model performance indices.
Figure 7. Case I: Variation of parameter range along the number of generations. W: weight parameter; B: bias parameter; I, H and O: input, hidden and output nodes, respectively; subscript i corresponds to the ith node in the respective layer, e.g. WI1H2 indicates a weight connection between 1st input and 2nd hidden node.
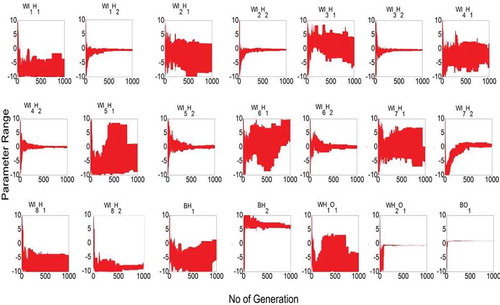
Figure 8. Case II: Variation of parameter range along the number of generations. See for explanation of notation.
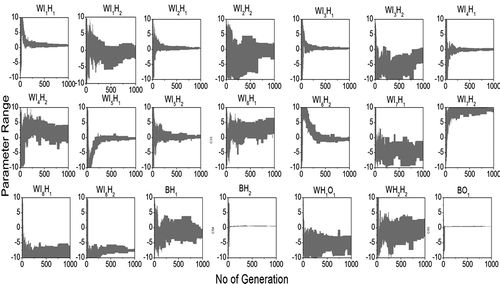
Table 3. Uncertainty indices estimated for Case I and Case II. POC: percentage of coverage; AW: average width.