Figures & data
Figure 1. Problem design. PCA – Principal Component Analysis, PM – Penman-Monteith, ETo – Reference evapotranspiration
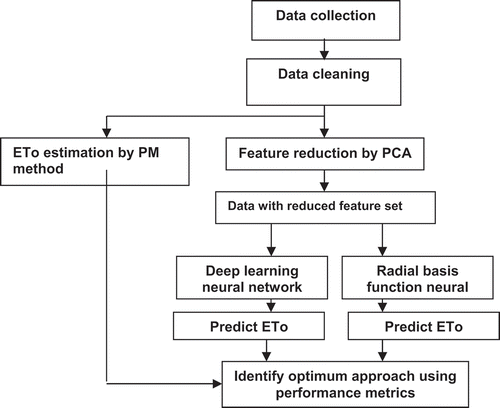
Table 1. Model scenario. See for attributes
Table 2. Statistical description of data model employed
Table 3. Attribute weights on selected principal components (PC)
Table 4. Scenario of the reduced-features data model
Table 5. Parameter optimization of the radial basis function neural network (RBFNN) architecture. MSE: mean squared error. Best values are indicated in bold
Table 6. Variation of mean squared error (MSE) with the number of convolution layers (conv) and fully connected (full) layers of the convolutional neural network (CNN). Bold formatting indicates the optimum number of layers
Table 7. Variation of the mean squared error (MSE) with the number of neurons in the fully connected layer of the convolutional neural network (CNN). Bold formatting indicates the optimum number of neurons
Table 8. Parameter values of the convolutional neural network (CNN) used in this study
Table 9. Performance metrics for the artificial neural network (ANN) methods used in this study. DLNN: deep learning neural network; MAE: mean absolute error; RBFNN: radial basis function neural network; RMSE: root mean square error
Table 10. Time taken to build the artificial neural network (ANN) methods. DLNN: deep learning neural network; RBFNN: radial basis function neural network
Table 11. Comparison of the artificial neural network (ANN) methods by regression coefficient, R2. DLNN: deep learning neural network; RBFNN: radial basis function neural network