Figures & data
Table 1. Statistics of the catchment descriptors
Figure 1. Land cover of Africa with the boundaries of the 314 catchments used in this study. MODIS land cover classes were combined into simpler classes (all types of forest are considered in the “Forest” class), and some minor and insignificant classes (urban, wetlands, snow/ice) were not added to the legend
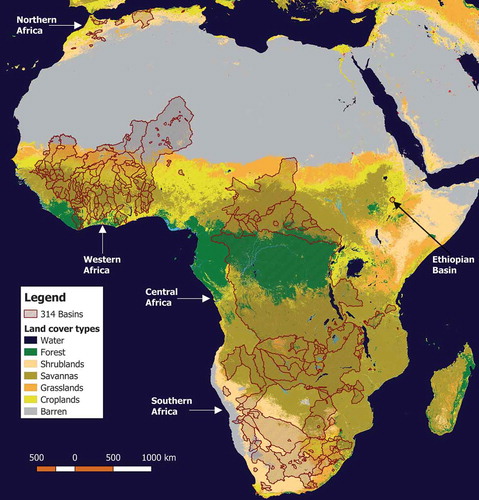
Figure 2. Average annual precipitation for each of the 314 African catchments used in this study. The large spatial variability of precipitation within and across regions can be noted
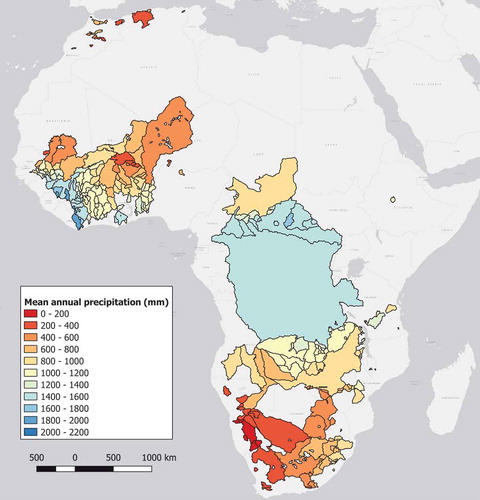
Figure 3. Calibration KGE for the 314 catchments. Three catchments have −0.25 < KGE < 0; 30 catchments have 0 < KGE < 0.4; 32 catchments have 0.4 < KGE < 0.6; 96 catchments have 0.6 < KGE < 0.8; and 156 catchments have KGE > 0.8
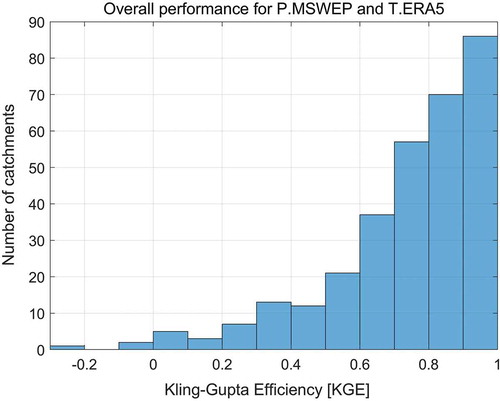
Figure 4. Spatial distribution of the calibration KGE values of the 314 catchments over Africa. Note that the larger catchments typically see better calibration KGE values than smaller catchments, except for the Niger River which is one of the worst in the dataset (KGE of −0.08)
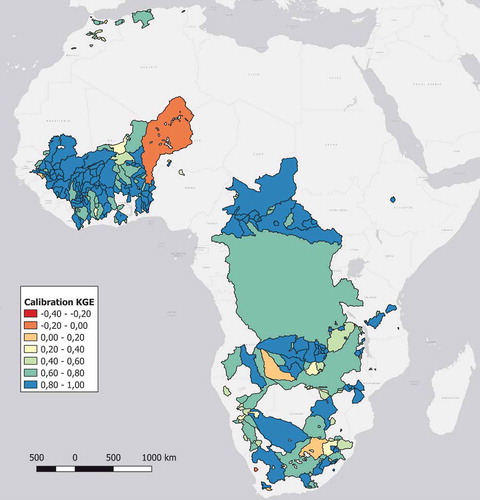
Table 2. Calibration KGE for different catchment size categories
Figure 5. KGE of multiple donor averaging generated hydrographs using the IDW approach for SP (top panel) and PS (bottom panel). Note that improvements plateau at approximately four or five donors for both methods. The x-axis indicates the number of donors that were weighted (Wt1 = one donor, Wt2 = two donors, etc.). The MLR box plot indicates the performance of the MLR method over the study domain and is identical in both panels
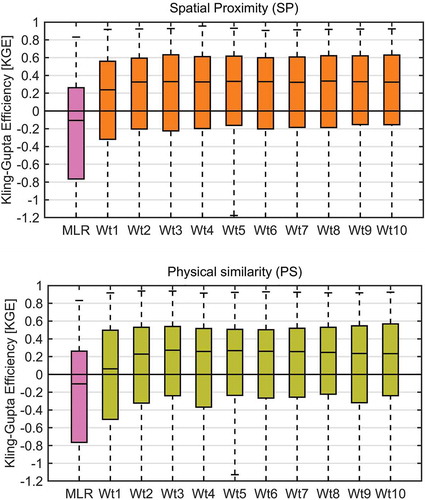
Figure 6. Spatial distribution of the three regionalization methods’ behaviour for the 314 catchments. Each dot’s color represents the ratio between the regionalized (Wt5) and the calibrated (Cal) KGE score for a given pseudo-catchment. The SP and PS regionalization KGE reflects that obtained by using the 5-donor weighted average
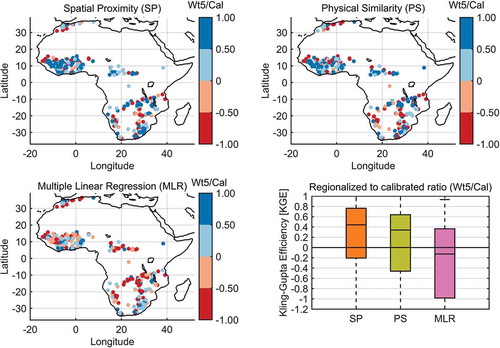
Figure 7. Catchments removed from the list of donors following the skill-based filtering. The three panels present the case with all donors (314 donors, left panel), with the KGE > 0.6 filter (250 donors, centre panel) and with the KGE > 0.8 filter (156 donors, right panel). Note that all North Africa and many Southern African catchments are removed with KGE > 0.8
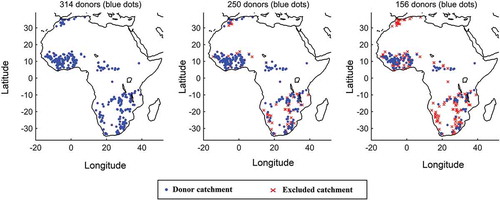
Figure 8. Spatial proximity (SP) regionalization KGE with skill-based filtering; row (a) regionalizing on all 314 pseudo-ungauged basins, row (b) regionalizing only on the subset of pseudo-ungauged basins that also pass through the filter (regionalizing only on well-calibrated pseudo-ungauged basins), and row (c) using all catchments as donors but regionalizing only on the “well-calibrated” catchments. The left column represents the base case (using all donors on all targets, and all three graphs are therefore identical), the centre column applies the first filter (KGE > 0.60) and the right column applies the second filter (KGE > 0.80). Titles indicate the number of donors and pseudo-ungauged targets used. Each panel has 5 box-plots: “Cal” is the calibration KGE for comparison purposes, “Near_1” is the regionalization KGE using the nearest single donor catchment, “Near_5” is the regionalization KGE using only the 5th nearest donor catchment, “Wt5” is the weighted 5-donor KGE and “Wt5/Cal” represents the Wt5/Cal ratio to scale the results as a function of the maximum possible attainable skill (i.e. the calibration skill)
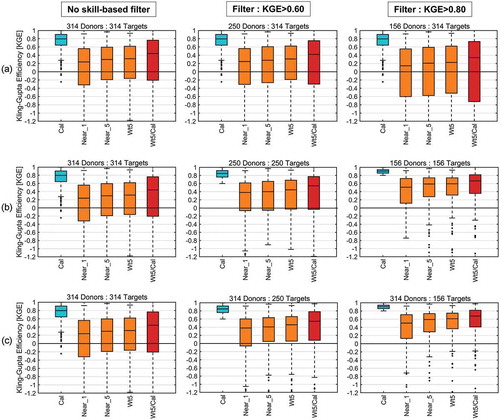
Figure 10. Same as but for the multiple linear regression (MLR) regionalization method. Each panel presents the calibration KGE, the MLR KGE and the ratio of MLR/Calibration
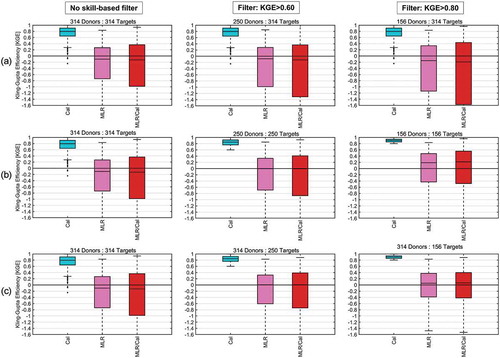
Table 3. Correlation coefficients between regionalization KGE and the catchment descriptors used in regionalization
Table 4. Average distance (in kilometers) between the ungauged catchment and its nearest donor based on the filtering level for both the SP and PS methods