Figures & data
Figure 1. Schematic of adiabatic, and non-adiabatic heating curves during the heating (coil on) and cooling (coil off) phases of a heat experiment. Common fitting time-frames to calculate SARv include: Time-Rise, Box-Lucas, Steady State, and Decay.
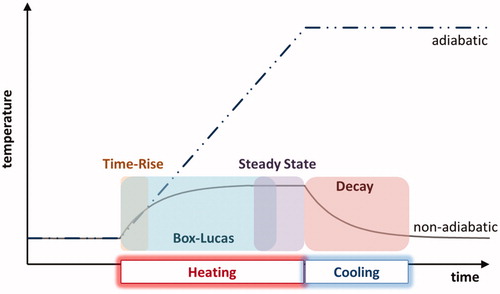
Figure 2. Effect of variation in SARv and RMSE using Box-Lucas and Time-Rise fitting as a function of SARv. Red circles indicate higher variation of SARv and RMSE caused by measurements performed in a sub-optimal heating range. The blue square indicates the range with a consistent SARv despite fitting method.
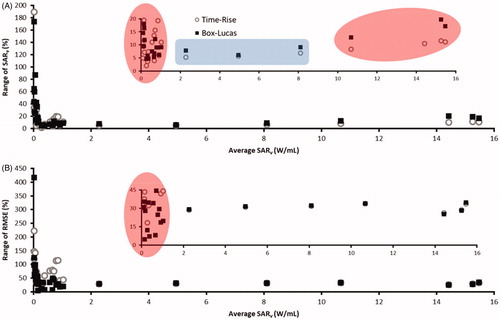
Figure 3. Evaluation and start time impact on SARv and RMSE. Plots A and B show the impact of evaluation time on SARv and RMSE when the start time is selected at 5s after heating. The SARv values are compared with the average SARv across all 12 fitting scenarios. A higher impact in both variation of SARv and RMSE is observed with Time-Rise fitting. Figure 3C evaluates the percentage difference in SAR between (1) SARv calculated excluding the first 5 s and (2) SARv calculated including t = 0-5 in the selected data range. Similarly, 3D evaluates the percentage difference in RMSE between these two cases. In this case, Box-Lucas fitting is observed to have larger variations in both SARv and RMSE than variations observed with Time-Rise fitting.
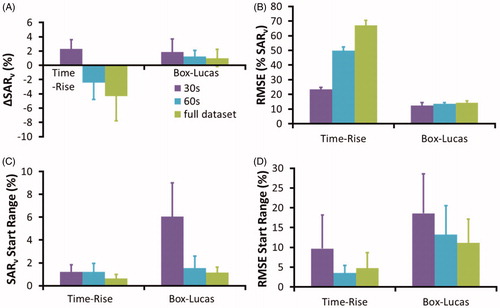
Figure 4. Repeatability of SARv measurement. Plot A shows the standard deviation of the replicate measurements as a function of evaluation time (using 5s start time) for SARv ≤ 0.1 W/mL. Plot B right shows the standard deviation of the replicate measurement as a function of evaluation time for SARv ≥ 0.1 W/mL. An increase in variation is observed at lower SARv. Furthermore, the R2 optimization results in more variable results than a statistically set evaluation time.
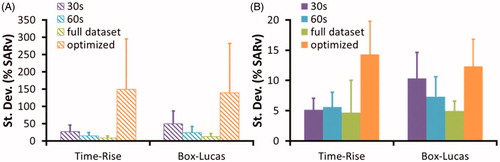
Table 1. Recommended parameters to reportTable Footnotea.
Table 2. Recommended parameters to report.