Figures & data
Table 1. Number of recurrences within patients for all 4 recurrent infections.
Table 2. Simulation results for the SML estimator and CSML estimator using a small (Z=10,000) Sobol point set.
Table 3. Simulation results for the SML estimator and CSML estimator using a large (Z=60,000 ) Sobol point set.
Table 4. Simulation results for the GHQ estimator.
Table 5. Joint model fixed parameter estimates (Est.) and standard errors (Std. err.) for the post kidney transplant data.
Figure 1. History and predicted probabilities of experiencing events for two patients. The dotted lines in the marker figures denote the fixed marker trajectories, that is, zero random effects. The continuous lines are the predicted marker trajectories. For the predictions, the probability of experiencing a particular infection is given. In addition, the sum of the infected no/yes probabilities is the probability that the patient has not dropped out of the study. (a) Patient without events and marker measurements until t=1 and (b) Patient with events and marker measurements until t=1.
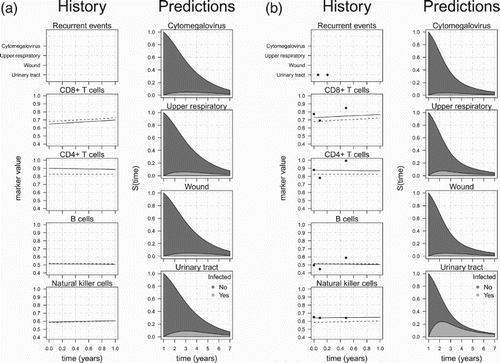