Figures & data
Table 1. Selected match and training GPS files—their modalities, drills and drill descriptions along with the number of GPS files, average number of observations (seconds) and corresponding average time per GPS file for each drill in minutes and seconds.
Figure 1. State distributions, with their parameter estimates and corresponding 95% confidence intervals, and stationary state probability plots for the five-state HMM fitted to the training data and the match data. For turning angle distributions, refers to an angle concentration parameter inversely related to variance. There was no heart rate data available for the match data, hence the absence of the stationary state probability plot in Panel (b).
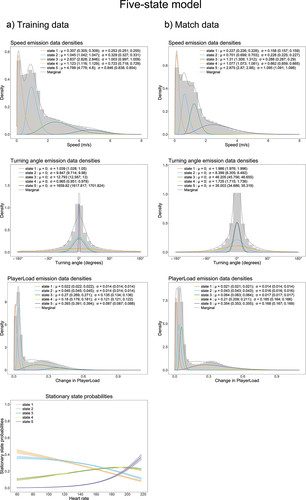
Figure 2. Stationary state probability plots for the five-state HMM, with proportion of match elapsed and score difference as transition probability covariates, fitted to the match data.
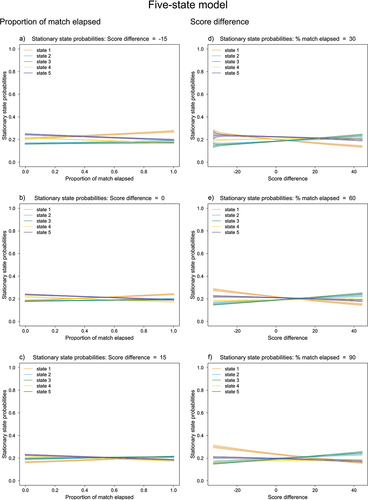
Figure 3. Proportion of time spent in each state for the five-state HMM, across all players for each training modality and the match and predicted match data.
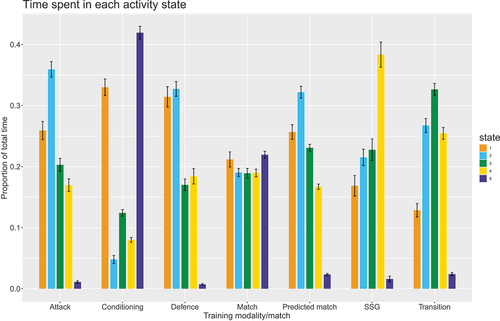
Figure 4. Cumulative time spent in each state (a) and player GPS files (b) for 5-state HMM fitted to training data.
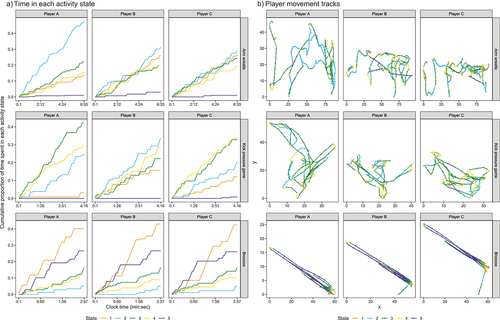
Figure 5. Cumulative time spent in each state (a) and player GPS files (b) for 5-state HMM fitted to match data. For display purposes, only the first 500 observations (500 seconds) of the GPS files are included.
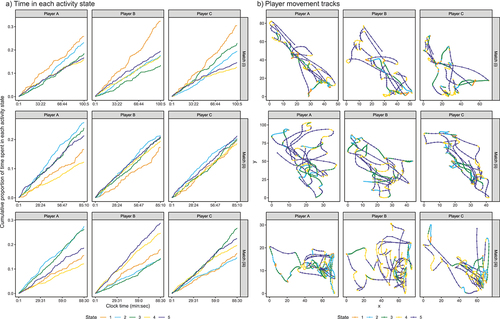
Table A1. AIC values for fitted models.
Figure A1. Examples of (a) GPS les with good quality that were retained and (b) GPS les with poor quality that were removed after visual inspection.
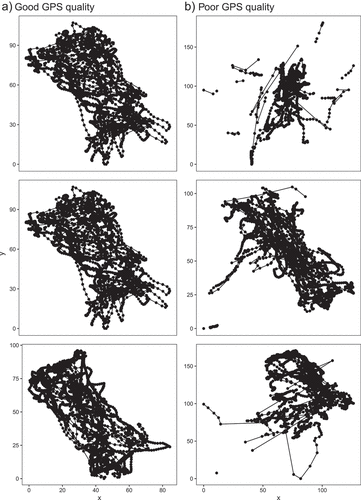
Figure A2. State distributions, with their parameter estimates and 95% confidence intervals for those estimates, and stationary state probability plots for the three-state HMM fitted to the training data (Panel a) and the match data (Panel b). For turning angle distributions, σ refers to an angle concentration parameter inversely related to variance. There was no heart rate data available for the match data, hence the absence of the stationary state probability plot in Panel b.
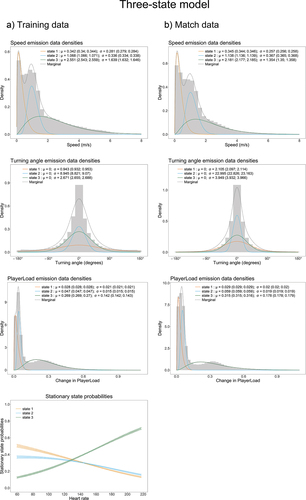
Figure A3. Stationary state probability plots for the three-state HMM, with proportion of match elapsed and score difference as transition probability covariates, fitted to the match data.
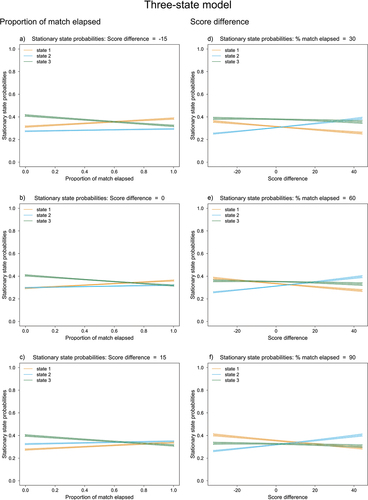
Figure A4. Proportion of time spent in each state for the three-state HMM, across all players for each training modality and for the match and predicted match data. The predicted match file was obtained by using the training model with the overall mean value for the heart rate covariate to predict the Viterbi sequence for each match data player’s GPS file.
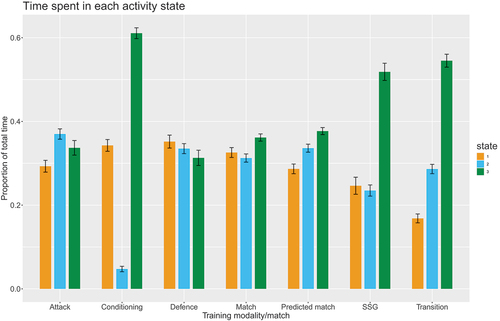
Figure A5. Cumulative time spent in each state (a) and player GPS files (b) for 3-state HMM fitted to training data.
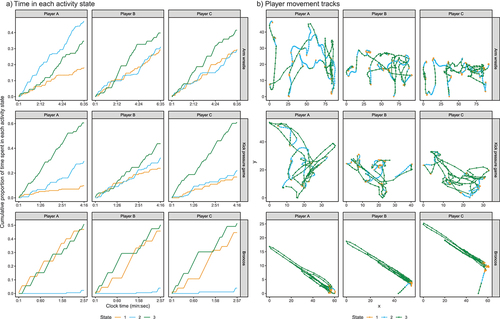