Figures & data
Figure 1. Histologic changes in inferior lacrimal gland following Concanavalin A
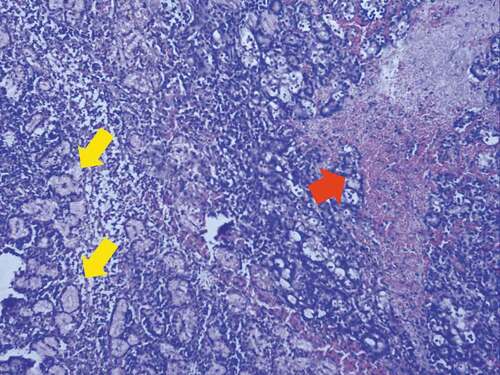
Table 1. Induction of DED in the three study groups
Figure 2. Principal Component Bi-Plots for surgical model of DED
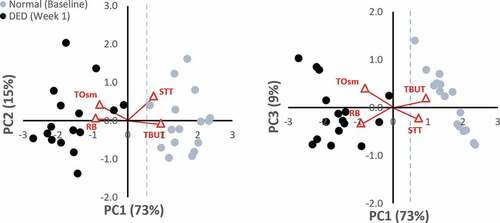
Table 2. Spearman correlation coefficients between DED parameters one week after its induction
Table 3. Relative contribution of the variables to principal components (%)
Figure 3. Variance for the surgical model of DED. The eigenvalues and explained variation for each of the four principal components (PC) is shown. The red line at the top depicts the cumulative variability explained, with the variability of each successive PC being added to that of the preceding one, reaching 100% with PC4. The majority of total variation (>70%) is explained by the first component
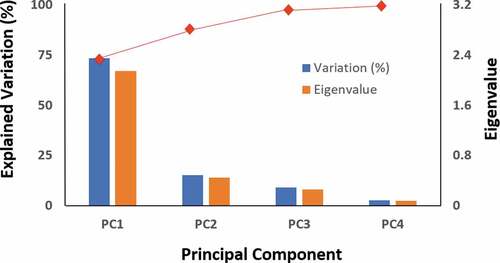
Figure 4. Principal Component Bi-Plots for the ConA groups
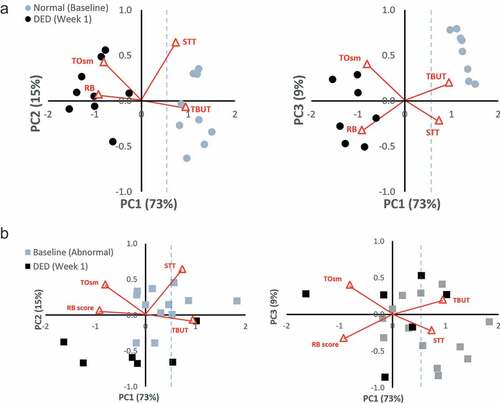
Table 4. P values for individual clinical test parameters and PC scores