Figures & data
FIG. 1 Normalized cumulative weight distributions of penetrating particles predicted by the Calvert theoretical model and optimally fitted by lognormal distributions.
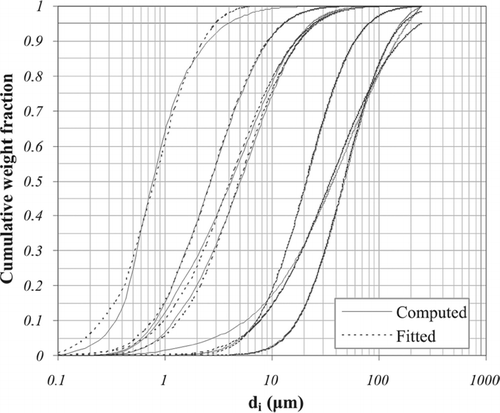
FIG. 2 (a) Nomographs based on the Calvert formulation, for Bf = 1.4, yielding the output particle distribution parameters G c d pα and lnσ p as function of the input particle distribution parameters G c d mα and lnσ g . (b) Nomographs based on the Calvert formulation, for Bf = 1.6, yielding the output particle distribution parameters G c d pα and lnσ p as function of the input particle distribution parameters G c d mα and lnσ g . (c) Nomographs based on the Calvert formulation, for Bf = 1.9, yielding the output particle distribution parameters G c d pα and lnσ p as function of the input particle distribution parameters G c d mα and lnσ g . (d) Nomographs based on the Calvert formulation, for Bf = 2.3, yielding the output particle distribution parameters G c d pα and lnσ p as function of the input particle distribution parameters G c d mα and lnσ g . (e) Nomographs based on the Calvert formulation, for Bf = 3, yielding the output particle distribution parameters G c d pα and lnσ p as function of the input particle distribution parameters G c d mα and lnσ g . (f) Nomographs based on the Calvert formulation, for Bf = 4, yielding the output particle distribution parameters G c d pα and lnσ p as function of the input particle distribution parameters G c d mα and lnσ g . (g) Nomographs based on the Calvert formulation, for Bf = 6, yielding the output particle distribution parameters G c d pα and lnσ p as function of the input particle distribution parameters G c d mα and lnσ g . (h) Nomographs based on the Calvert formulation, for Bf = 16, yielding the output particle distribution parameters G c d pα and lnσ p as function of the input particle distribution parameters G c d mα and lnσ g .
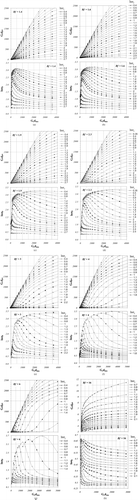
FIG. 3 Algorithm for generating best-fit lognormal distribution parameters d p and σ p from cumulative weight fraction series computed through the use of Calvert and Yung et al. formulations.
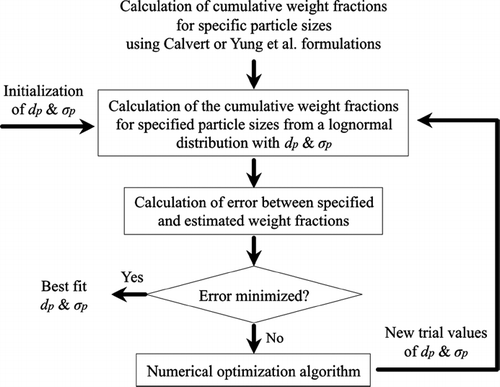