Figures & data
Table 1. Experimental matrix highlighting the relevant experiments from the FLAME-IV campaign used in this study.
Figure 1. Experimental setup for gas-particle partitioning experiments during the FLAME-IV campaign.
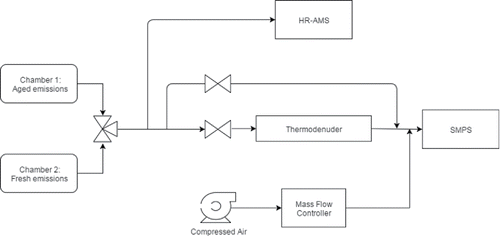
Figure 2. Equilibration profiles for (a) all emissions data, (b) fresh emissions data, and (c) aged emissions data from all experiments after applying the optimization routine. The accommodation coefficients (α) obtained for each case are shown on the respective graphs. Upon binning the scattered data, we see that it follows the behavior of the general dynamic response (GDR) curve.
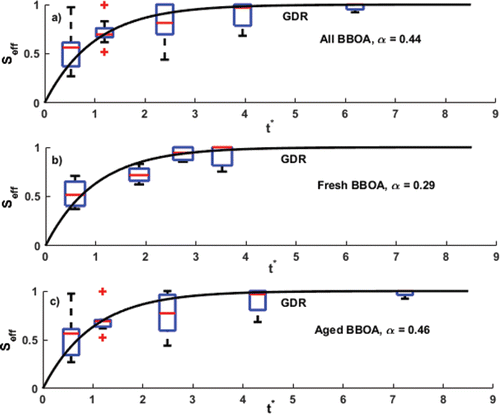
Figure 3. The binned data from the entire experimental matrix plotted with t* calculated from different values of α. This figure shows the significant deviation from the universal dynamic response with equilibration time scales calculated from logarithmically spaced accommodation coefficient values.
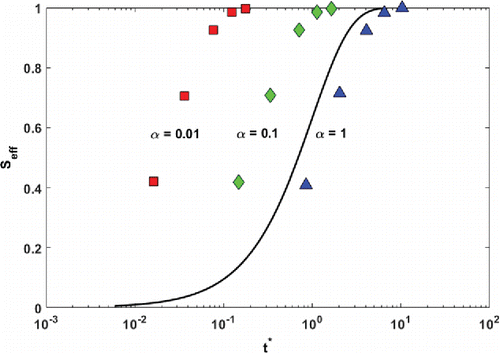
Table 2. Mass accommodation values found in this study for biomass burning emissions for different assumptions on molar mass and gas phase diffusion coefficient.
Figure 4. Fractional difference between dynamic and equilibrium cases simulating dispersion of a biomass-burning plume for a range of values of the mass accommodation coefficient α. After about an hour the dynamic and equilibrium cases converge and the relative difference is small regardless of the assumed value of α, but for the best-estimate value (α = 0.44) at no time does the error in the equilibrium partitioning calculation exceed 7%.
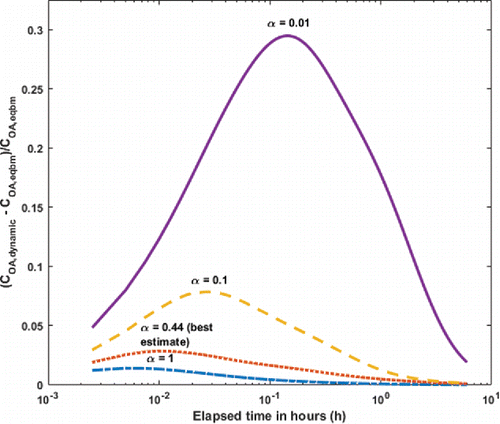