ABSTRACT
Number of attempts have been made in the past to improve the generalization performance of artificial neural networks (ANN). Internal adjustment in ANN deals with tuning of various parameters like learning rate, activation function, etc. Majority of activation functions that exist in the literature are transcendental in nature. In this paper, a novel parametric algebraic activation (PAA) function has been proposed. The function PAA is a generalized function of which Elliott activation function is a special case. PAA is a family of S-shaped curves and satisfies all the important properties of activation functions. This activation is employed on resilient propagation algorithm (RPROP) learning algorithm. Comparative performance evaluation with the widely known activation functions has been carried out on various benchmark datasets taken from University of California Irvine (UCI) machine learning repository. Comparative performance evaluation in terms of number of epochs and testing error of the proposed PAA has been made with the standard activation functions. It has been observed that decrease in number of epochs and testing error for the proposed PAA in RPROP is highly statistically significant for most of the UCI datasets when compared with standard activation functions. Thus incorporating PAA in RPROP can make it more powerful for classification.
ACKNOWLEDGMENTS
We are thankful to the director Dr G.R.C. Reddy, NITGOA for providing constant encouragement throughout this research work. We are also thankful to reviewers for their valuable suggestions.
DISCLOSURE STATEMENT
No potential conflict of interest was reported by the authors.
Additional information
Notes on contributors
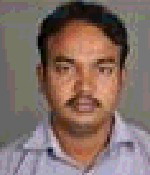
K. V. Naresh Babu
K. V. Naresh Babu is presently working as an assistant professor in Computer Science and Engineering Department at NIT Goa. He has successfully graduated with MTech and PhD degrees from Indian Institute of Technology Delhi. He has also worked as a senior research associate at Evalueserve Pvt Ltd. His research interests include big data analytics and machine learning. He has published several research publications in national and international conferences and journals.
E-mail: [email protected]
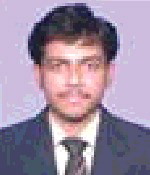
Damodar Reddy Edla
Damodar Reddy Edla received BSc degree from Kakatiya University in 2004, MSc degree from University of Hyderabad in 2006 and MTech and PhD degrees in Computer Science and Engineering from Indian School of Mines, Dhanbad in 2009 and 2013, respectively. His research area is data mining and wireless sensor networks. He is currently Head of the Computer Science and Engineering Department, National Institute of Technology, Goa, India. He has more than 20 research publications in reputed international journals and conferences.
E-mail: [email protected]