ABSTRACT
The proportional-integral-derivative (PID) is widely used in industrial control systems due to its simplicity. For multi-input multi-output (MIMO) systems, choosing the PID gain values is a difficult task especially that in most applications system parameter variations and changes in operating conditions occur. Thus, there is necessity to find parameters adjustment method in which PID gains should be adapted to handle such changes. This paper is concerned with the design of a multivariable adaptive PID (APID) controller in which recursive least square (RLS) algorithm is used as an adaptation mechanism. The RLS algorithm, on the contrary of its usual application as an identification method, is used in the proposed controller to update the PID gains online forcing the system to behave like a desired reference model. Unlike other techniques, the proposed multivariable APID controller has the advantage that it does not impose restrictions on the system structure such as being stable, square, minimum phase, nor almost restrict positive real. Since stability is a vital issue in the evaluation of control systems, therefore stability analysis of the proposed approach is developed using Lyapunov stability theory. Comparative simulation results demonstrate the superiority of the proposed multivariable APID controller over another two different controllers when applied to MIMO systems especially for unstable systems when the controlled systems suffer from parameter variations or uncertainties.
DISCLOSURE STATEMENT
No potential conflict of interest was reported by the authors.
Additional information
Notes on contributors
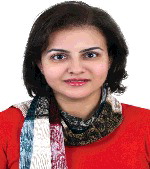
Rania A. Fahmy
Rania A. Fahmy received BSc degree in electronics and communications in 2002, MSc degree in 2009 and PhD in 2016 in systems and control engineering all from Faculty of Engineering, Cairo University, Egypt. She is currently a lecturer in the Egyptian Nuclear and Radiological Regulatory Authority (ENRRA). Her research interests include adaptive control and control of nuclear reactors.
E-mail: [email protected]
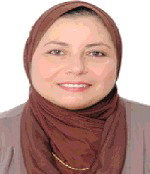
Ragia I. Badr
Ragia I. Badr obtained her BSc degree with distinction and honours in 1979 from Electronics and Communications Department, Faculty of Engineering, Cairo University, MSc degree in systems and control Engineering in 1982 and PhD degree in systems and control engineering in 1987 from Cairo University. In 1987, she was appointed as an assistant professor in Electronics and Communications Department, Faculty of Engineering, Cairo University. She is currently a professor at the same department and her research areas include robust control, neuro-fuzzy control, and adaptive-non-linear control.
E-mail: [email protected]
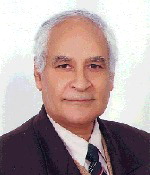
Farouk. A. Rahman
Farouk A. Rahman holds BSc degree in 1970, MSc degree in 1973, and PhD degree in 1977 in nuclear engineering from Faculty of Engineering, Alexandria University, as well as a bachelor of laws degree in 1991 from the Faculty of Law and Bachelor of Commercial Science in 1987 from Faculty of Commerce, Ain-Shams University. He is the Head of the Nuclear Reactor Operation Licensing Group, and member of the IAEA Transport Safety Standards Committee (TRNSSC). His research interests are in the safe operation, quality assurance, and regularity inspections of nuclear research reactors.
E-mail: [email protected]