Abstract
For any industry, an important part of quality control is the detection and identification of defects of the products. During the manufacturing process, a wide range of defects occur on or in the products. These defects have an impact on its esthetics and functionality of the end-product. Their ability to compete in the market and the manufacturing costs are to be compromised if they are not detected properly. In many industries, defect detection is done manually by a few trained inspectors. The inspection results of this kind are highly subjective, and their measurements are seldom repeatable. The availability of fast and specialized computing hardware nowadays has facilitated the emergence of image processing algorithms to do online and real time quality inspections. As a result, many industries, such as textiles, steel, semiconductors, wood, leather, etc., are looking forward to machine-vision based inspection systems to address the issues pertaining to manual inspection. A comprehensive literature review of defects Identification, Localization, and Classification methods proposed to implement in such imaging systems is presented in this paper. The advantages and disadvantages of these suggested and implemented these methods are compared. The performance metrics used for the evaluation of these methods are also presented. In addition, the utilization of Optimization Techniques and Artificial Neural network structures in these systems is investigated. This review can help the researcher engaged in the area of automatic texture inspection systems to investigate the texture identification, localization, and classification methods.
Additional information
Notes on contributors
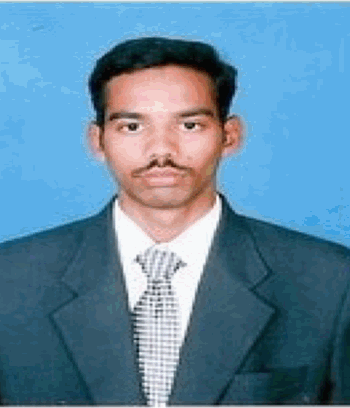
Md. Khwaja Muinuddin Chisti
Md Khwaja Muinuddin Chisti received his ME from Andhra University, Visakhapatnam. Presently, he is pursuing his Ph.D. at JNT University, Kakinada. He is working as an assistant professor in the EECE Department, GITAM deemed to be University, Visakhapatnam, Andhra Pradesh, India. His area of interest is Digital image processing. Corresponding author. Email: [email protected], [email protected]
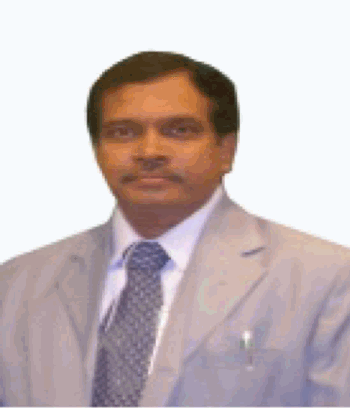
S. Srinivas Kumar
Srinivas Kumar Samayamantula is working as a professor in the ECE Department, UCEK, JNTUK, Kakinada, Andhra Pradesh, India. He received his M.Tech. from JNTU, Hyderabad, India. He received his PhD from E&ECE Department, IIT, Kharagpur. He has 35 years of experience in teaching and research. He has published more than 125 research papers in national and international journals and also in proceedings of reputed conferences. His research interests are digital image processing, computer vision, and the application of artificial neural networks and fuzzy logic to engineering problems. Email: [email protected]

Gandikota Prasad
Prasad Gandikota is retired scientist-F & deputy director, Central Electronics Engineering Research Institute (CEERI), Chennai, India. He received MTech from Andhra University, Visakhapatnam. He received PhD from ECE Department, Anna University, Chennai. He has 23 years of R&D in CEERI and 16 years of experience in teaching. His research interests are in the areas of fuzzy logic and neural networks, design and development of the microprocessor and computer-based systems, embedded systems, image processing, industrial automation and instrumentation & controls. Email: [email protected]