Figures & data
Table 1. Catchment characteristics used in this study and their range of values for the 90 Ontario basins (modified after Samuel et al. Citation2011).
Figure 2. Flowchart of methodology showing the four regionalization techniques – inverse distance weighted (IDW), multi-layer perceptron (MLP), support vector machine (SVM), and counter propagation neural network (CPNN) – used to transfer the parameters of two hydrologic models (McMaster University Hydrologiska Byråns Vattenbalansavdelning [MAC-HBV] and Sacramento Soil Moisture Accounting [SAC-SMA]) from gauged to ungauged watersheds considering two scenarios of classified and unclassified watersheds using non-linear principal component analysis (NLPCA), CNLPCA (compact NLPCA), and self-organizing map (SOM) techniques.
![Figure 2. Flowchart of methodology showing the four regionalization techniques – inverse distance weighted (IDW), multi-layer perceptron (MLP), support vector machine (SVM), and counter propagation neural network (CPNN) – used to transfer the parameters of two hydrologic models (McMaster University Hydrologiska Byråns Vattenbalansavdelning [MAC-HBV] and Sacramento Soil Moisture Accounting [SAC-SMA]) from gauged to ungauged watersheds considering two scenarios of classified and unclassified watersheds using non-linear principal component analysis (NLPCA), CNLPCA (compact NLPCA), and self-organizing map (SOM) techniques.](/cms/asset/38d57234-9570-4bbb-b71e-a47f6bb04963/tcwr_a_1184590_f0002_b.gif)
Table 2. Parameters of McMaster University Hydrologiska Byråns Vattenbalansavdelning (MAC-HBV) and Sacramento Soil Moisture Accounting (SAC-SMA) models and optimized ranges (using particle swarm optimization [PSO] algorithm).
Figure 3. Schematic representation of the Sacramento Soil Moisture Accounting (SAC-SMA) model as used in this study showing the model state parameters used. Abbreviations are presented in Table . Arrows indicate fluxes between components and the streamflow at the watershed outlet is shown (adapted from Vrugt et al. Citation2006).
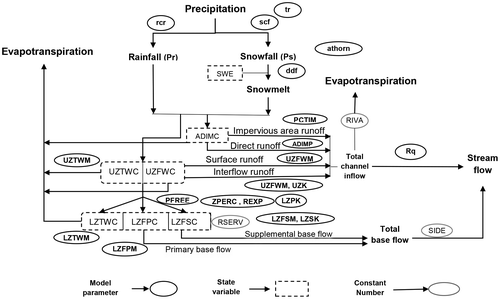
Figure 4. Box plots of NSE and VE values for simulated daily stream flows for 90 watersheds across Ontario using calibrated model parameters of (a) McMaster University Hydrologiska Byråns Vattenbalansavdelning (MAC-HBV) and (b) Sacramento Soil Moisture Accounting (SAC-SMA). These resulted from the following optimization algorithms: particle swarm optimization (PSO), shuffle complex efficiency (SCE), non-sorted genetic algorithm II (NSGA II), and Monte Carlo simulation for validation period 1986–1994. Nash Sutcliffe efficiency (NSE) values above 0.5 and volume error (VE) values between −0.1 and 0.1 are considered to be models with good performance.
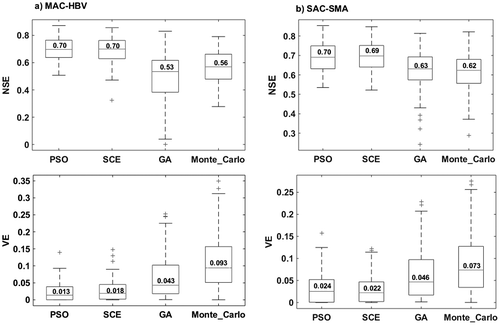
Table 3. Statistics of Nash Sutcliffe efficiency (NSE) values of estimated daily streamflow using McMaster University Hydrologiska Byråns Vattenbalansavdelning (MAC-HBV) and Sacramento Soil Moisture Accounting (SAC-SMA) models coupled with the following regionalization techniques: inverse distance weighted (IDW), multi-layer perceptron (MLP), counter propagation neural network (CPNN) and support vector machine (SVM). These were applied to unclassified watersheds (Unc) and classified watersheds with self-organizing map (SOM), non-linear principal component analysis (NLPCA) and CNPLCA (compact NLPCA) for the validation period from 1986 to 1994.
Table 4. Nash Sutcliffe efficiency (NSE) values of estimated daily baseflow using McMaster University Hydrologiska Byråns Vattenbalansavdelning (MAC-HBV) and Sacramento Soil Moisture Accounting (SAC-SMA) models coupled with the following regionalization techniques: inverse distance weighted (IDW), multi-layer perceptron (MLP), counter propagation neural network, (CPNN) and support vector machine (SVM). These are applied to unclassified watersheds (Unc) and classified watersheds with self-organizing map (SOM), non-linear principal component analysis (NLPCA) and CNPLCA (compact NLPCA) for the validation period from 1986 to 1994.
Table 5. Percentage of basins with deterioration more than −20% and improvement greater than 20% in (a) root mean square error (RMSE) of daily streamflow, (b) volume error (VE) of daily baseflow, (c) VE of daily peak flow, using the following classification techniques: non-linear principal component analysis (NLPCA), CNLPCA (compact NLPCA), and self-organizing map (SOM), applied prior to the following regionalization techniques: inverse distance weighted (IDW), multi-layer perceptron (MLP), counter propagation neural network (CPNN) and support vector machine (SVM), and on hydrologic models McMaster University Hydrologiska Byråns Vattenbalansavdelning (MAC-HBV) and Sacramento Soil Moisture Accounting (SAC-SMA).
Figure 5. Schematic maps of the spatial distribution of improvements in Root Mean Square Error (RMSE) and Volume Error (VE) of daily streamflow, low flow and peak flow regionalization using the counter propagation neural network (CPNN) technique on the McMaster University Hydrologiska Byråns Vattenbalansavdelning (MAC-HBV) model after (a) non-linear principal component analysis (NLPCA) and (b) self-organizing map (SOM) classification techniques. Small circles identify the basins with a consistent improvement of > 20% in daily mean, low and peak flow regionalization after watershed classification.
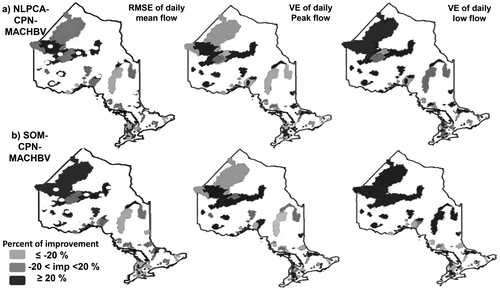
Figure 6. Schematic maps of the spatial distribution of improvements of Root Mean Square Error (RMSE) and Volume Error (VE) in daily streamflow, low flow and peak flow regionalization, using the counter propagation neural network (CPNN) regionalization technique on the Sacramento Soil Moisture Accounting (SAC-SMA) model after (a) non-linear principal component analysis (NLPCA) and (b) self-organizing map (SOM) classification techniques. Small circles identify the basins with a consistent improvement of > 20% in daily mean, low and peak flow regionalization after watershed classification.
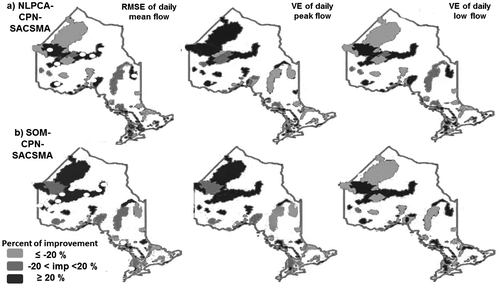
Figure 7. Observed and simulated streamflow using the following hydrologic models: (a) McMaster University Hydrologiska Byråns Vattenbalansavdelning (MAC-HBV) and (b) Sacramento Soil Moisture Accounting (SAC-SMA) models coupled with counter propagation neural network (CPNN) technique on unclassified (CPN-Unc) and classified watersheds using non-linear principal component analysis (NLPCA) (CPN-NLPCA) on three sample watersheds specified in Figure 1.
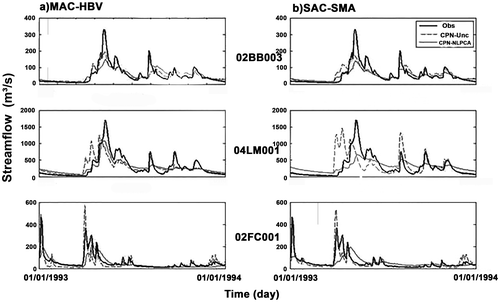
Figure 8. The hydrograph of mean monthly flow in northern, central and southern watersheds of Ontario.
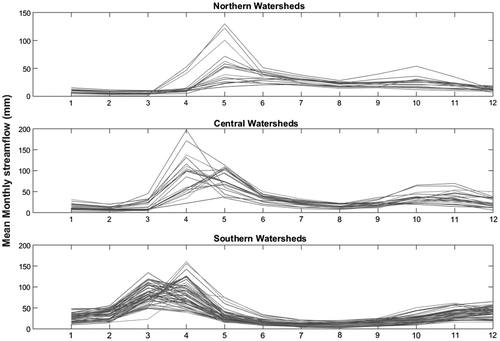
Figure 9. Spatial variability of percentage of land covered by: forest, lake, rapid drainage class, glacio-deposit, and glaciofluvial sediments and slope of FDC (Flow Duration Curve) in selected Ontario watersheds.
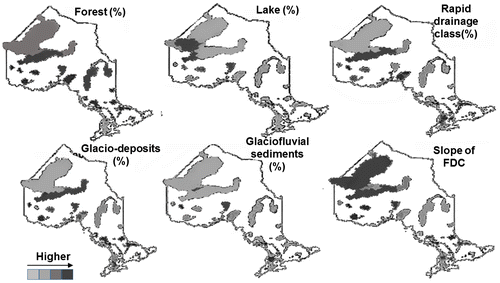