Figures & data
Table 1. Summary of the bathymetric LiDAR classification methods.
Figure 1. Test site: (a) Marco Island in Florida, USA and VQ-880-G point cloud data and (b) Samcheok in Gangwon-Do, South Korea and Seahawk point cloud data.
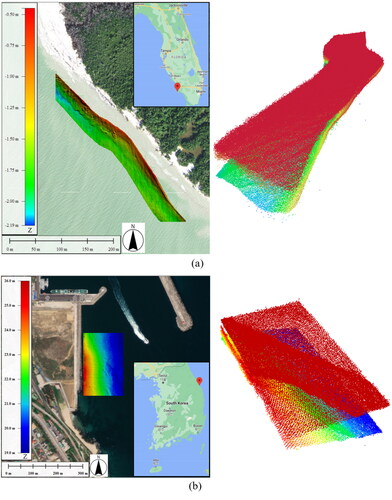
Table 2. Summary of the test datasets.
Figure 2. Examples of (a) waveform decomposition; (b) water levels; and (c) pseudo-waveform decomposition.
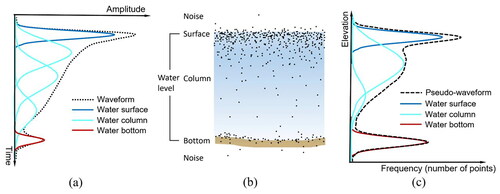
Figure 3. Key steps of the proposed workflow: (a) 2D grid cell structure generation; (b) point cloud that falls onto a single cell; (c) pseudo-waveform generation; (d) pseudo-waveform decomposition; (e) pseudo-waveform classification; and (f) classified point cloud.
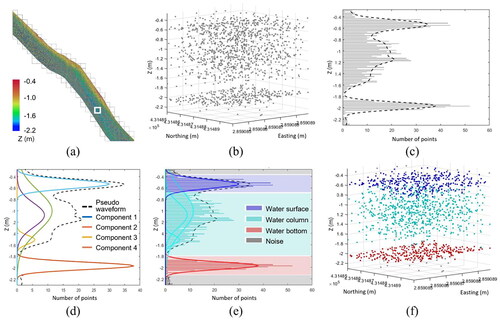
Figure 5. Iterative Gaussian decomposition of pseudo-waveform by estimating the potential peaks: (a) original peak detection; (b,c) estimation of the potential peak through Gaussian curve fitting; (d) pseudo-waveform decomposition result.
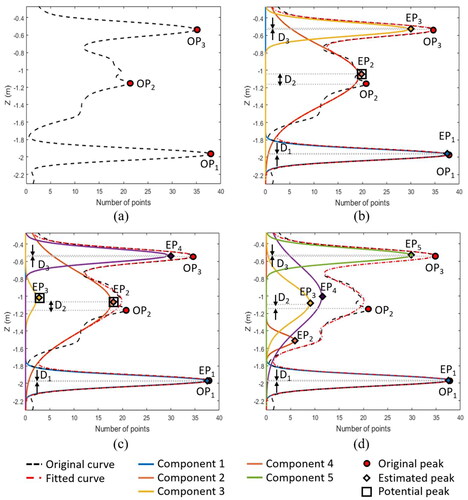
Figure 6. Examples of the classification bound determination with (a) four components; (b) two separate components; (c) two intersecting components.
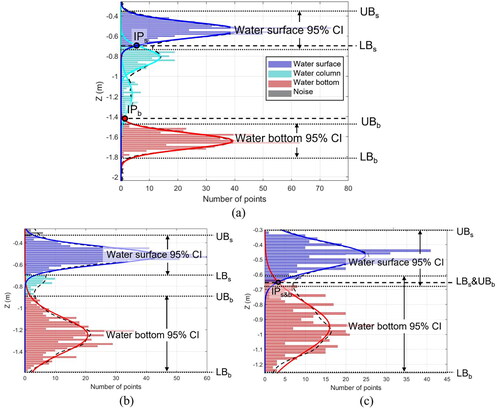
Table 3. Test variables for sensitivity analysis.
Table 4. Top 10 combinations of parameters in descending order of overall accuracy for VQ-880G data.
Table 5. Bottom 10 combinations of parameters in ascending order of overall accuracy for VQ-880G data.
Figure 7. Sensitivity analysis that accounts for both water bottom and surface with respect to three parameters: (a) cell size; (b) smoothing filter size; (c) Z threshold.
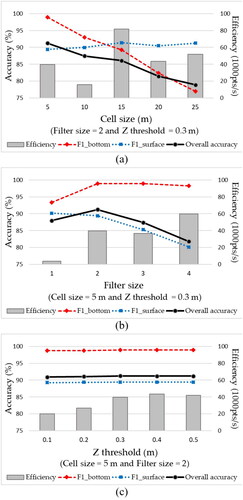
Table 6. Performance comparison of the water bottom classification methods for VQ-880G data.
Figure 8. Classified water bottom points of VQ-880G data: (a–e) close-up view of region #1; and (f–j) close-up view of region #2.
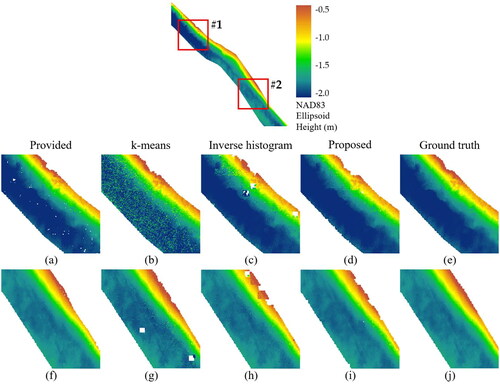
Figure 9. Examples of classification results by depth and water bottom type: (a) clear separation of the water bottom (depth < 1.7 m); (b,c) unclear separation of the water bottom (depth < 1.3 m); (d) very shallow water (depth < 0.7 m); (e) uneven water bottom (depth < 1 m).
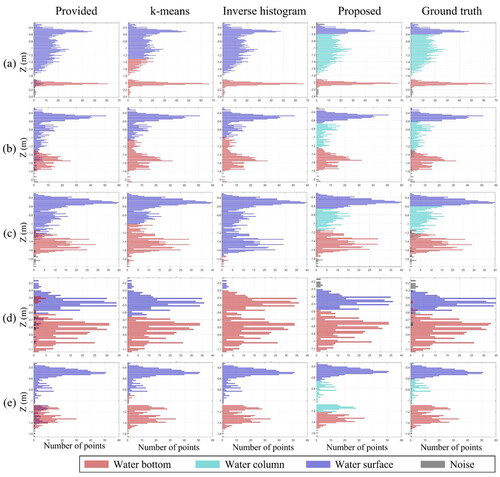
Table 7. Optimized parameters and accuracies of the classification result of Seahawk data.
Figure 10. Error distribution in the Z-direction (ΔZ) of Seahawk data: (a) classified water bottom points; (b) MBES data; (c) the difference map of ΔZ; (d) frequency histogram of ΔZ.
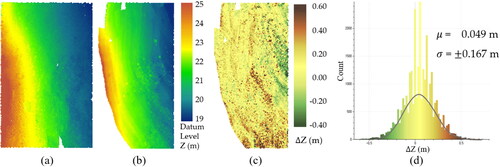
Table A1. Specifications for Riegl VQ-880G system.
Table A2. Specifications for Seahawk system.