Figures & data
Table 1. The Cityscapes dataset partition.
Table 2. Pretraining details.
Figure 10. Pre-training FPN, DeepLabV3, and DeepLabV3+ segmentation networks on GTAv synthetic dataset.
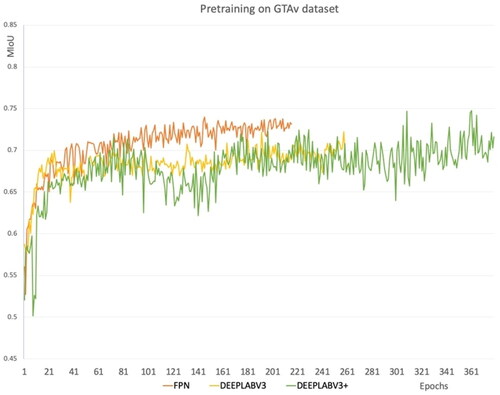
Table 3. Fine-tuning details.
Table 4. Percentage of region numbers from all of the dataset.
Table 5. Comparison of performance of the FPN network across various methods with different budgets (MIoU).
Table 6. Performance of our method on six underrepresented classes (MIoU).
Table 7. The comparison of performance of the DeepLabV3 network across various methods with different budgets (MIoU).
Table 8. The comparison of performance of the DeepLabV3 network across various methods with different budgets (MIoU).
Figure 16. The segmentation models are trained on all of the Cityscapes dataset, containing 2975 images. The results are compared to IoU for validation.
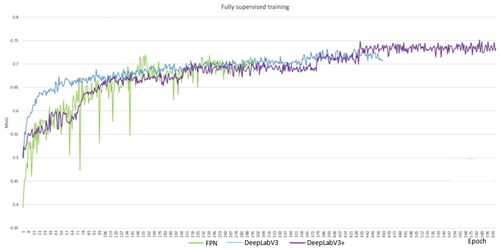
Table 9. The performance of supervised and FWA RALis methods across three networks (MIoU).
Table 10. The performance of baselines and RALis method across three networks with 8% budget of all data (MIoU).