Figures & data
Fig. 1 Estimated optimal shrinkage intensities for and
(MP) as function of concentration ratio c > 1 and dimension p = 300.
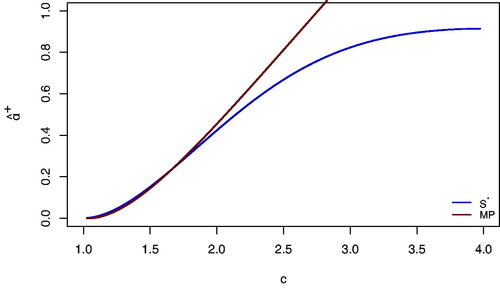
Fig. 2 Estimated optimal shrinkage intensities for and
(MP) as function of dimension p for c = 1.5 (left) and c = 2 (right).
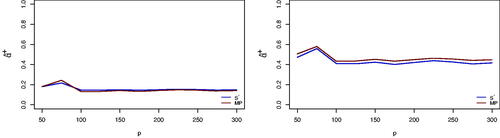
Fig. 3 The asymptotic optimal shrinkage intensity as a function of c for the calibration criteria (i)-(ii) from Proposition 2.1 (left to right).
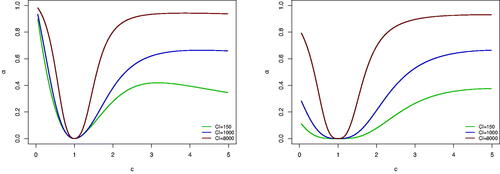
Fig. 4 The relative losses for the portfolios based on the optimal shrinkage estimator, the traditional estimator and the equally weighted portfolio as a function of c for the calibration criteria (i)-(ii) from Proposition 2.1 (left to right). The dimension is set to p = 100 and the condition index is set to 1000.
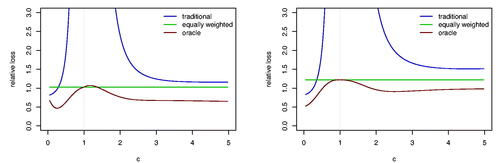
Fig. 5 The relative losses for the portfolios based in the optimal shrinkage estimator, the traditional estimator and the equally weighted portfolio as a function of the dimension p for 0.2 (top left), 0.5 (top right), 0.8 (bottom left), 2 (bottom right). The condition index is set to 1000 and the mean-variance calibration criteria is used.
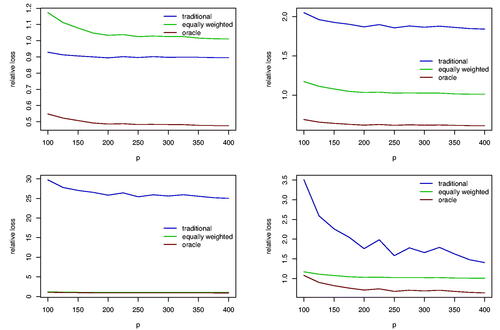
Table 1 Performance of traditional, bona-fide, the benchmark portfolios (LWQuEST— Ledoit and Wolf 2017b, LWAnalytical— Ledoit and Wolf 2020, KZ - Kan and Zhou Citation2007) and the target portfolios for the mean-variance calibration criteria.
Table 2 Performance of traditional, bona-fide, the benchmark portfolios (LWQuEST - Ledoit and Wolf 2017b, LWAnalytical - Ledoit and Wolf 2020, KZ - Kan and Zhou Citation2007) and the target portfolios for the minimum variance calibration criteria.
Fig. 6 The bona-fide shrinkage intensities for the first 100 assets (alphabetic order) using the equally weighted target portfolio and the mean-variance calibration for c = 0.2, 0.5, 0.8 and 2. Above - bona fide, below - bona fide ridge (see formula (2.33)).
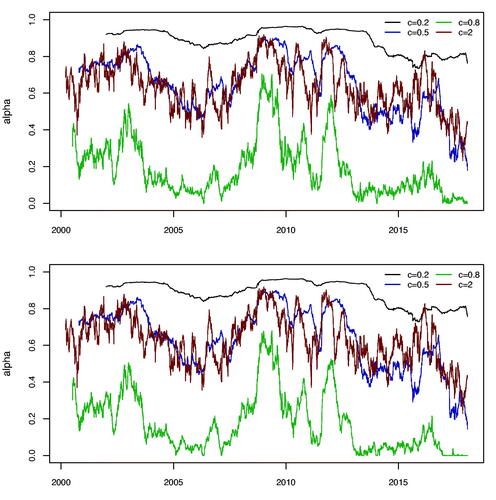