Figures & data
Table 1. COVID-19 relevant ICF categories and levels.
Figure 1. Overview of the annotation data divided into non-COVID-19 and COVID-19 notes for each annotated ICF category. Each category is differentiated in levels (0–4 and in the case of Walking & Moving and Exercise Tolerance 0–5).
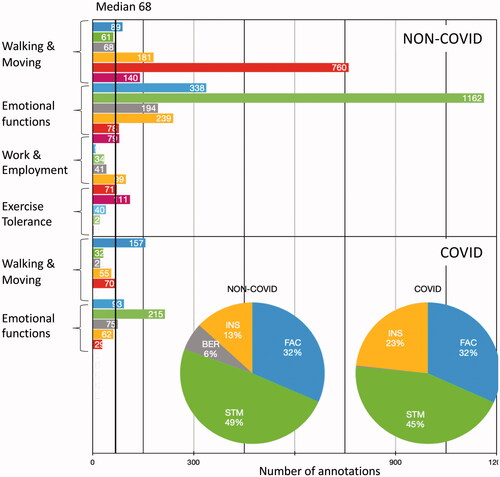
Figure 2. Distribution of level annotations for Walking & Moving (FAC score) across COVID-19 and non-COVID-19 data.
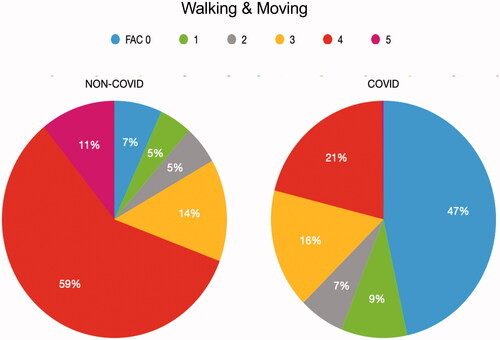
Table 2. Performance of the ICF category classification.
Table 3. Best models regression analysis for each relevant scoring level.
Data availability statement
The data that support the findings of this study are available on request from the corresponding author, CGM. The data are not publicly available due to containing information that could compromise the privacy of research participants.