Figures & data
FIGURE 1 Sequences from our industrial environment dataset. Object tracking as well as activity recognition is extremely challenging due to occlusions, low resolution, and high intraclass and low interclass variance. The first two rows depict two different activities that are executed during the production cycle: their resemblance is so high, that they would be difficult to distinguish even for the human eye; the third row shows some example frames of occlusions, outliers, sparks, abnormalities, etc. (Figure is provided in color online.)
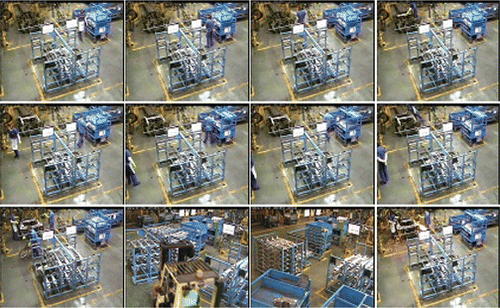
FIGURE 2 HMM-based fusion approaches for streams. Symbols s and o stand for states and observations, respectively. The first index indicates the stream and the second the time. (Figure is provided in color online.)
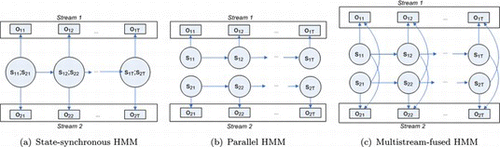
FIGURE 3 Schematic overview: The neural network-based rectification mechanism is examined under two different approaches (corresponding to the green and red paths, respectively). The green approach rectifies the fused result produced by the fused HMM, whereas the red one performs streamwise rectification and in the sequel the rectified streams are fused (RDFHMM). (Figure is provided in color online.)
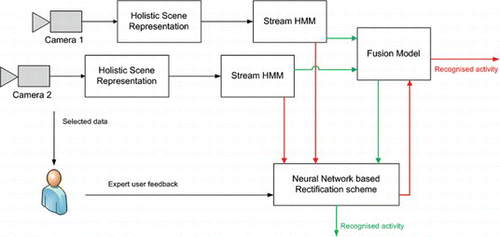
FIGURE 4 Depiction of workcell together with the position of the cameras and racks #1–5. (Figure is provided in color online.)
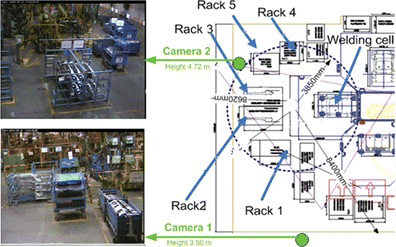
TABLE 1 Results Obtained from Dataset-1 and Dataset-2 Using (1) Individual HMMs to Model Information from Stream 1 (HMM1); (2) Individual HMMs to Model Information from Stream 2 (HMM2); (3) State-Synchronous HMMs (SYNC); (4) Parallel HMMs (PARAL); and (5) Multistream-Fused HMMs (MULTI) with (a) Gaussian and (b) Student's -distribution as Observation Likelihood
FIGURE 5 Confusion matrices from dataset-1 for (a) individual HMM for camera 1, (b) individual HMM for camera 2 and (c) multistream-fused HMM, using Student's t -distribution. (Figure is provided in color online.)
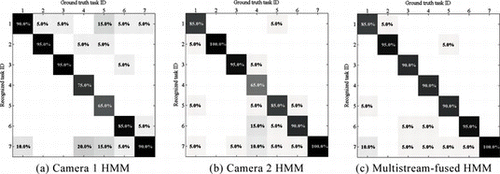
TABLE 2 Results Obtained from Dataset-1 and Dataset-2 After Applying the Rectification Mechanism (RM) Using (1) Individual HMMs to Model Information from Stream 1 (HMM1); (2) Individual HMMs to Model Information from Stream 2 (HMM2); (3) State-Synchronous HMMs (SYNC); (4) Parallel HMMs (PARAL); (5)Multistream-Fused HMMs (MULTI); and (6) Rectification-Driven Fused HMM (RDFHMM) with (a) Gaussian and (b) Student's -distribution as Observation Likelihood
FIGURE 6 Classification error % with and without the rectification mechanism for all experimental setups: 1. HMM1-Gauss, 2. HMM1-Student-t, 3. HMM2-Gauss, 4. HMM2-Student-t, 5. SYNC-Student-t, 6. SYNC-Student-t, 7. PARAL-Gauss, 8. PARAL-Student-t, 9. MULTI-Gauss, 10. MULTI-Student-t, 11. RDFHMM-Gauss, 12. RDFHMM-Student-t, (11 & 12 have no corresponding nonrectified setup). (Figure is provided in color online.)
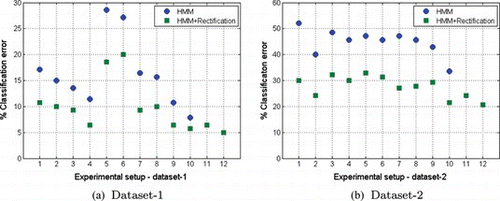
FIGURE 7 Improvement ratio % in terms of error for all experimental setups: 1. HMM1-Gauss, 2. HMM1-Student-t, 3. HMM2-Gauss, 4. HMM2-Student-t, 5. SYNC-Gauss, 6. SYNC-Student-t, 7. PARAL-Gauss, 8. PARAL-Student-t, 9. MULTI-Gauss, 10. MULTI-Student-t, (above mentioned 11 & 12 have no corresponding non-rectified set-up therefore no improvement rate can be calculated). (Figure is provided in color online.)
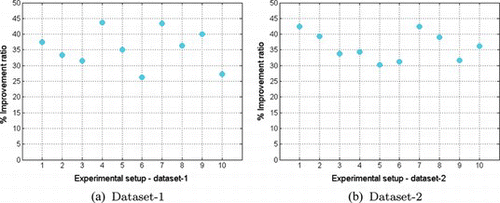