Figures & data
TABLE 1 An Exemplary Decision Table with a Binary Decision
FIGURE 1 An attribute-clustering tree for the decision table from , obtained by applying the agglomerative nesting algorithm in combination with the direct discernibility dissimilarity function.
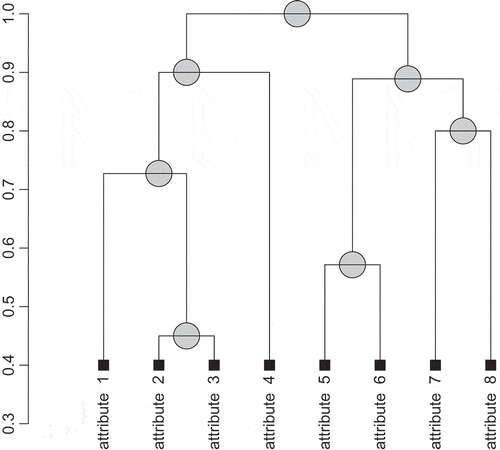
TABLE 2 Average Computation Times of 100 Reducts for Permutations Produced Using Different Clusterings
TABLE 3 Average Sizes of 100 Reducts Computed for Different Clusterings
TABLE 4 Average Minimal Sizes among 100 Reducts Computed for Different Clusterings
TABLE 5 Distribution of Attributes in Clusterings into 10 Groups Using the Agnes Algorithm
FIGURE 2 A visualization of the clustering trees for five different gene dissimilarity measures, which were cut at a height corresponding to the division into 10 groups.
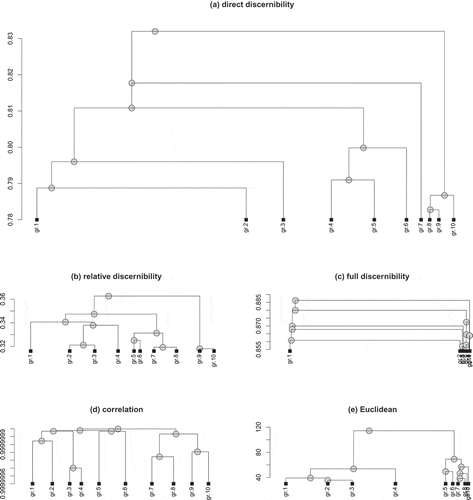
TABLE 6 Performance of the Ordered Reducts (OR) and Ordered Reducts with Diverse Attribute Drawing (OR-DAD) Algorithms with and without Attributes from the Group 1 of the Direct Discernibility Clustering into 10 Groups (Results of 20 Independent Repetitions of the Experiment)
FIGURE 3 Average computation times, minimal and average sizes, and average maximal overlap of reducts computed using the RR-DAS and OR-DAS algorithms based on direct discernibility. Plots correspond to different settings of the attribute sample size used in every iteration of the algorithms.
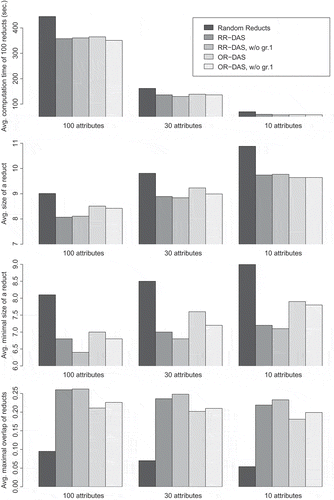
TABLE 7 Stability of an Attribute Selection Using Different Reduct Computation Algorithms