Figures & data
Figure 4. Variational auto encoder model where observable variable and its correspondent latent
are distributed on Gaussian distribution
and
, respectively.
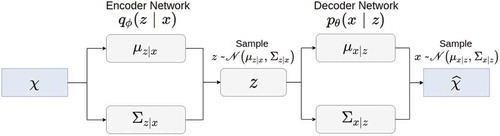
Figure 6. AutoEncoding variational inference for aspect discovery. As illustrated, the yellow block and
is corresponded to the document-topic and topic-word distributions which is described in , respectively. Meanwhile,
and
are additional blocks which play an important role in the aspect discovery task.
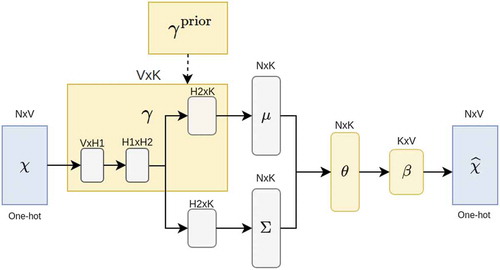
Table 1. Discovered aspects (bold text indicates seed words).
Figure 7. Example of constructing the square loss term. Firstly, every rows in the matrix is normalized via softmax function. After that, a submatrix is constructed by choosing only set of words in the normalized matrix
such that this word must also exists in a given
matrix. Finally, the prior loss is computed via the Euclidean distance between this submatrix and
.
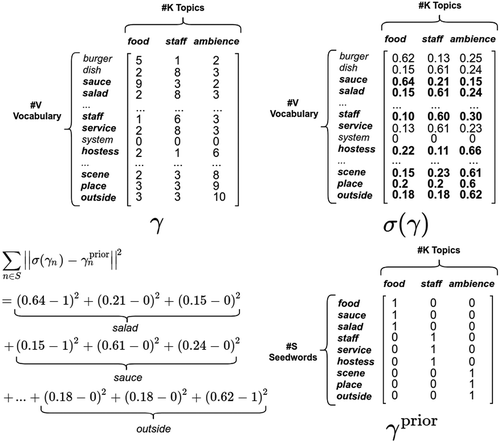
Figure 8. Autoencoding variational inference for joint sentiment/topic modeling where ,
and
are the corresponding latent variables in JST graphical model (). Moreover, the yellow block which is used to mapping document
to latent variable
can be represented as any modern classification deep neural network.
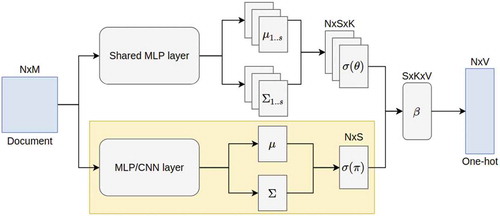
Table 2. AVIAD and WLDA seedwords.
Table 3. Statistics for IMDB and Yelp datasets.
Table 4. Topics extracted by AVIAD and WLDA.
Table 5. IMDB Topics extracted by AVIJST and JST.
Table 6. Yelp Topics extracted by AVIJST and JST.
Table 7. Sentiment words discovered.
Table 8. Aspect identification results.
Table 9. Accuracy on test set for IMDB.
Table 10. Accuracy on test set for Yelp.