Figures & data
Figure 1. Block Diagram depicting two phases of proposed land usage identification technique using aerial images with feature level fusion of TSBTC and Sauvola thresholding with ensemble of machine learning algorithms
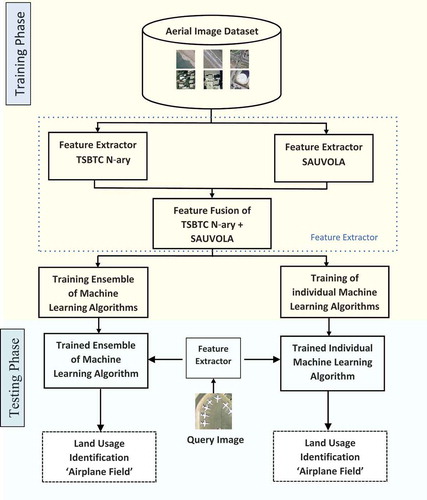
Figure 2. Sample aerial images of 21 categories of land usage from UC Merced land use dataset (Yang and Newsam Citation2010)
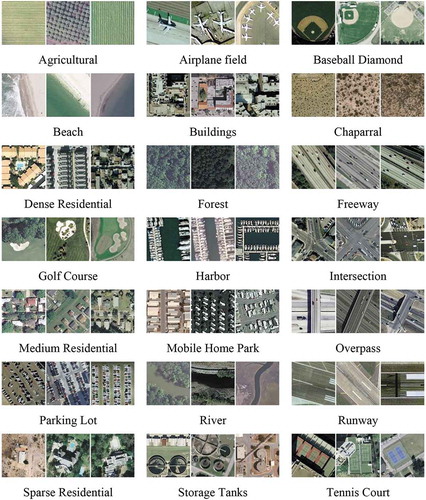
Figure 3. Percentage accuracy-based performance appraise of variations of TSBTC N-ary global features for respective machine learning algorithms and ensembles in proposed land usage identification technique
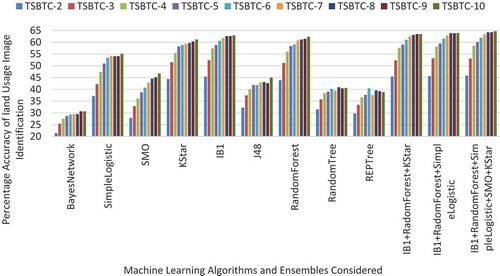
Figure 4. Percentage accuracy-based performance appraise of considered machine learning algorithms and ensembles for respective TSBTC N-ary global feature vector extraction method variants in proposed land usage identification technique
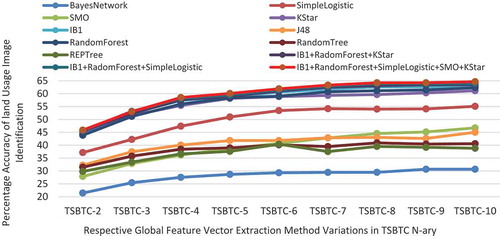
Figure 5. Percentage accuracy-based performance appraise of machine learning algorithms and ensembles for Sauvola thresholding based local feature vector extraction method in proposed land usage identification technique
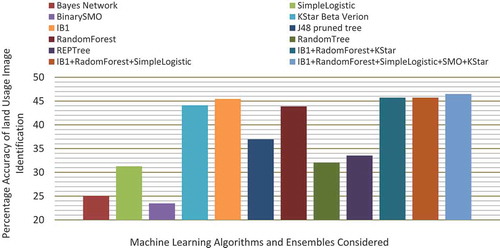
Figure 6. Performance Appraise of considered ensembles of machine learning algorithms for respective Feature Level Fusion combinations of local features of Sauvola and global features in proposed land usage identification technique
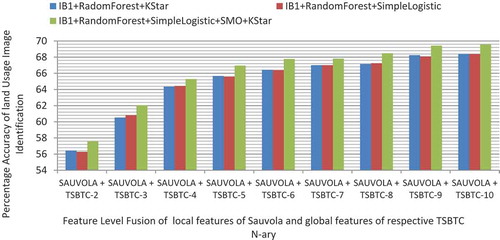
Figure 7. Performance Appraise of Feature Level Fusion of local features of Sauvola and global features of respective TSBTC N-ary for considered ensembles of machine learning algorithms in proposed land usage identification technique
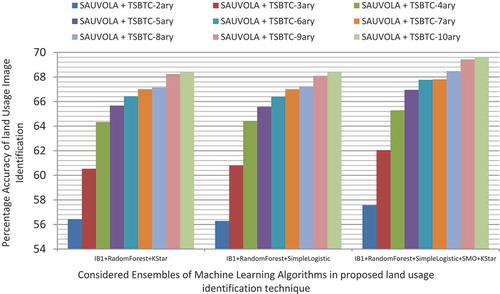
Figure 8. Percentage accuracy-based performance appraise of various feature extraction methods considered as TSBTC N-ary, Sauvola and feature level fusion of TSBTC N-ary and Sauvola for individual machine learning algorithms and respective ensembles in proposed land usage identification technique
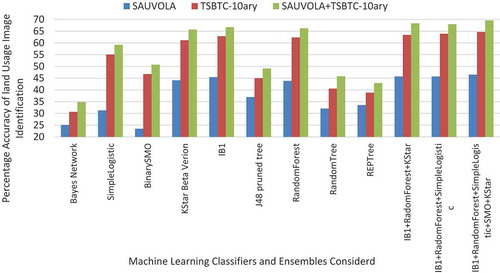
Figure 9. Matthews Correlation Coefficient (MCC) based performance appraise of various feature extraction methods considered as TSBTC N-ary, Sauvola and feature level fusion of TSBTC N-ary and Sauvola for individual machine learning algorithms and respective ensembles in proposed land usage identification technique
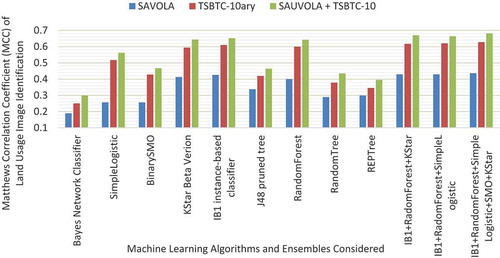
Figure 10. F Measure based performance appraise of various feature extraction methods considered as TSBTC N-ary, Sauvola and feature level fusion of TSBTC N-ary and Sauvola for individual machine learning algorithms and respective ensembles in proposed land usage identification technique
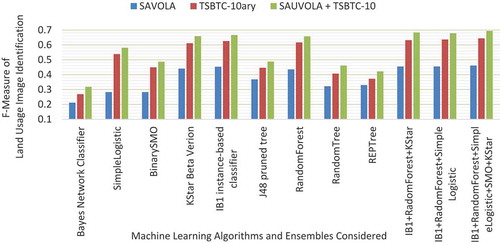