Figures & data
Figure 1. Periodicities of the traffic accidents series. (a) Number of accidents depending on day of the week. Weekends present less number of accidents. (b) Number of accidents for each month. August seems to be safer. (c) Number of accidents depending on hour of the day. In this case we have the most clear difference
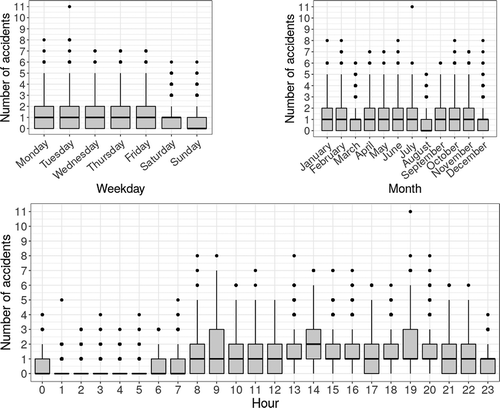
Table 1. Values used for each hyper-parameter. is the dimension of the latent space. The remaining variables were presented in Section 3 or are commonly used parameters
Table 2. Performance for to
traffic accident regression
Figure 4. Forecasting performance (MAE and bias) of the different models by timestep together with the calculated distributions
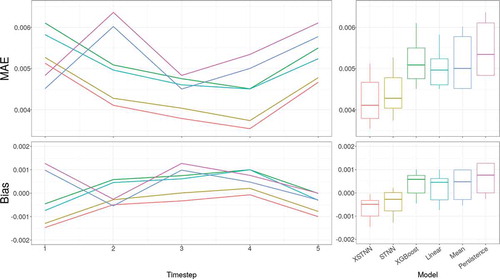
Figure 5. A practical example of the operation of both networks, XSTNN and STNN, for the same situation. From p.m. to
p.m. on a Wednesday
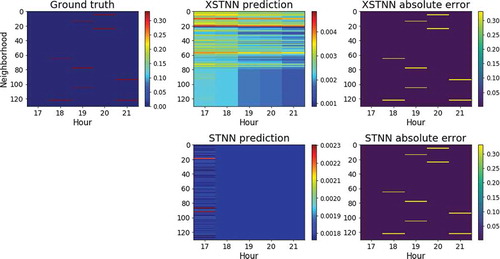