Figures & data
Figure 1. Tuta absoluta’s life cycle and its damage to tomatoes. (a) Four stages of T. absoluta’s life cycle. (b) Tomato leaf with T. absoluta mines. (c) Severe damage on tomato field. (d) Damaged tomato fruits in the field. (e) Damaged tomato fruit on the market

Figure 2. Experimental setup in a field. (a) A nethouse. (b) Researcher and an agricultural expert performing infestation in Arusha and Morogoro fields
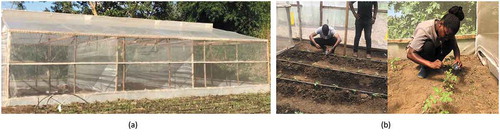
Table 1. Dataset distribution
Table 2. Train/test set splits
Table 3. Training time
Figure 8. Training and validation loss curve for Mask RCNN. Loss graph for (a) Mask RCNN-ResNet50, (b) Mask RCNN-ResNet101, (c) Mask RCNN-Resnet50 with augmentations, and (d) Mask RCNN-Resnet101 with augmentations
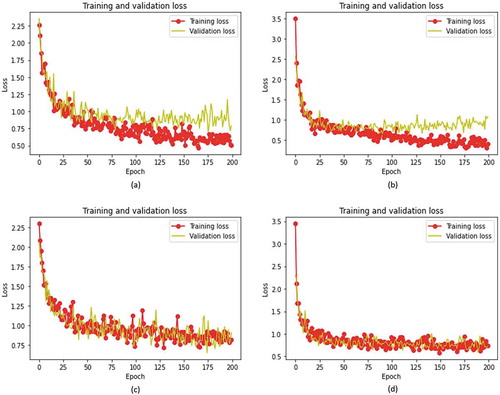
Figure 10. The evaluation metrics results for the semantic segmentation model. (a) IoU for U-Net. (b) Dice Coefficient for U-Net
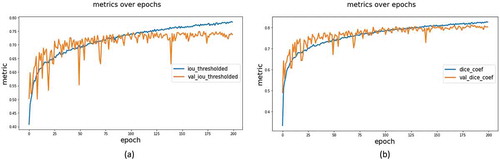
Table 4. The mAP (primary metric) values of the tomato images obtained by different detection methods