Figures & data
Figure 1. Statistical representation of label noise (Frenay and Verleysen Citation2013). NCAR (a) (Noisy completely at random) is an acronym for noisy completely at random. NAR (b) stands for noisy at random. NNAR (c) stands for noisy not at random.
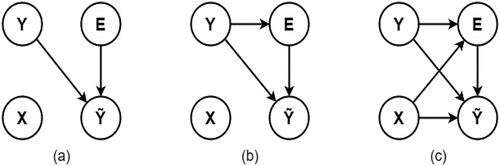
Figure 2. (a) Bangladesh geographical location. (b) High-resolution satellite image of target Region. (c) Targeted marine region.
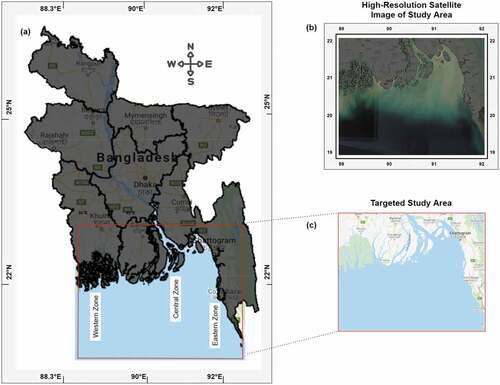
Figure 3. Image labeling and band extraction (a) 12-band satellite image. (b) Labeling with google earth engine. (c) Generating shapefile by QGis Software. (d) Adding shapefile as the 13th band to the original image.
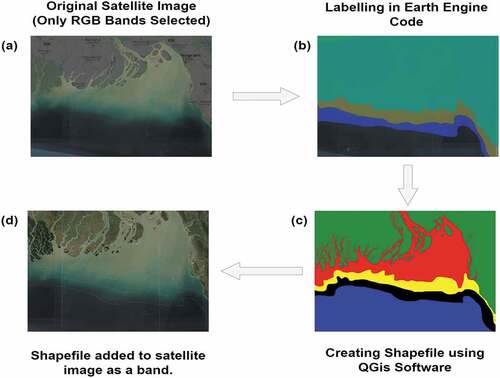
Figure 4. Preparing dataset from the satellite image. (a) 4-band satellite image (4th band is a label). (b) Stacked layers of an image. (c) The first three layers (bands) are separated as the image. (d) 4th band is separated as a mask.
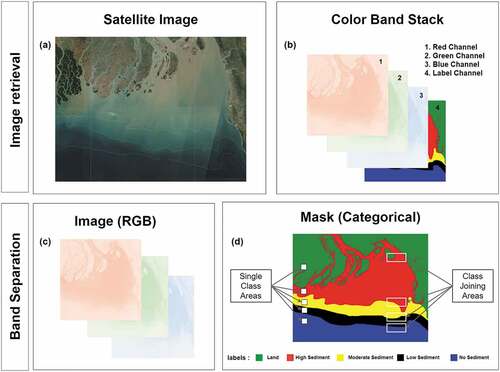
Table 1. Performance measurement for four-year dataset.
Table 2. Dice coefficient, pixel accuracy, and loss for complete random label noise.
Table 3. Dice coefficient, pixel accuracy, and loss for rotational label noise.
Table 4. Dice coefficient, pixel accuracy, and loss for label flip noise.