Figures & data
Figure 1. Variational autoencoder for classification and regression model. Note that “Predictor” can be a classification or regression network.
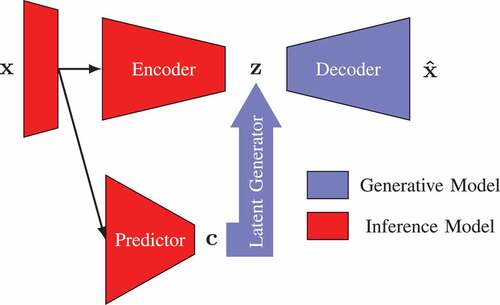
Table 1. False alarms for detecting OOD data in N-BaIot dataset.
Table 2. Classification accuracy and AUROC for detecting OOD data in gearbox dataset.
Table 3. AUROC for detecting OOD data in MNIST dataset.
Table 4. VAE for classification architecture in MNIST.
Table 5. VAE for regression architecture in AEBS.
Table 6. False alarms for detecting OOD data in AEBS.
Figure 6. Histogram of ground-truth distance of training dataset that excludes data ranging from to
.
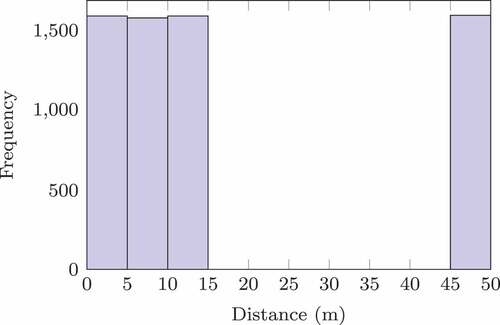