Figures & data
Table 1. A list of metal-organic frameworks with ultra-high Brunauer-Emmett-Teller (BET) surface area and pore volume.
Figure 1. (Colour online) Schematic representation of a few MOFs with high gas storage property. As an example, MOF-5 is known for high H storage capacity, and is composed of ZnO (light green), acting as the metal ‘node’ and while benzodicarboxylate serves as the ‘linker’ molecule. Most MOFs have acronymns and they are often named after the institute or place of origin. Reprinted with permission from Acta Crystallographica Section B [Citation30].
![Figure 1. (Colour online) Schematic representation of a few MOFs with high gas storage property. As an example, MOF-5 is known for high H2 storage capacity, and is composed of ZnO (light green), acting as the metal ‘node’ and while benzodicarboxylate serves as the ‘linker’ molecule. Most MOFs have acronymns and they are often named after the institute or place of origin. Reprinted with permission from Acta Crystallographica Section B [Citation30].](/cms/asset/df78c2a7-7a52-4e0a-8753-377890c946d7/gmos_a_1916014_f0001_oc.jpg)
Table 2. A list of metal-organic frameworks databases.
Figure 2. (Colour online) A typical machine learning workflow applied to discovery of MOFs. The orange-shaded or the inner region covers the three step process of building an ML model for material discovery (provided the features and training set are available) with the following steps: model selection, model training, and validation. The outer region, coloured in light red, covers the descriptor selection, dataset creation, and model testing parts of the process. This type of inner and outer region representation of the workflow helps us to understand the purpose of the inner region, which is to perfect the model given the dataset and descriptor via traversing these sub-processes: model selection, optimisation, and tuning. The outer region is more focused on handling the dataset and also its best representation in the form of descriptors.
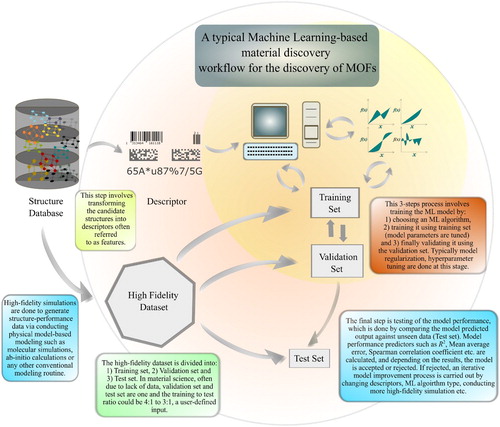
Figure 3. (Colour online) (a) Selectivity plot of Xe/Kr in the training set with RMSE data [Citation103], (b) Feature importance plot for all descriptors, (c) Selectivity plot of Xe/Kr in the test set with RMSE data, and (d) Distribution of simulated selectivities in the diverse training set compared with a randomly selected set from the dataset. Reprinted with permission from American Chemical Society.
![Figure 3. (Colour online) (a) Selectivity plot of Xe/Kr in the training set with RMSE data [Citation103], (b) Feature importance plot for all descriptors, (c) Selectivity plot of Xe/Kr in the test set with RMSE data, and (d) Distribution of simulated selectivities in the diverse training set compared with a randomly selected set from the dataset. Reprinted with permission from American Chemical Society.](/cms/asset/f67bffba-5ea2-4f49-91a9-9bfbc0f12a8c/gmos_a_1916014_f0003_oc.jpg)
Figure 4. (Colour online) A DT model for the CO uptake capacity higher than (a) 2 mmol/g, (b) 4 mmol/g of the 32,450 MOFs in the training data set. Each branch represents a QSPR score of 1 or 0 assigned to that MOF if it satisfies a descriptor threshold criteria. The final scores below are averaged QSPR scores [Citation48]. Reprinted with permission from European Journal of Inorganic Chemistry.
![Figure 4. (Colour online) A DT model for the CO2 uptake capacity higher than (a) 2 mmol/g, (b) 4 mmol/g of the 32,450 MOFs in the training data set. Each branch represents a QSPR score of 1 or 0 assigned to that MOF if it satisfies a descriptor threshold criteria. The final scores below are averaged QSPR scores [Citation48]. Reprinted with permission from European Journal of Inorganic Chemistry.](/cms/asset/52b15963-333b-4aa8-85f9-97c441ca5cb7/gmos_a_1916014_f0004_ob.jpg)
Figure 5. (Colour online) The top plot figure shows the log(frequency) with net H delivery capacity for all the databases [Citation107]; while the bottom figure is the net deliverable energy versus void fraction plot. Reprinted with permission from American Chemical Society.
![Figure 5. (Colour online) The top plot figure shows the log(frequency) with net H2 delivery capacity for all the databases [Citation107]; while the bottom figure is the net deliverable energy versus void fraction plot. Reprinted with permission from American Chemical Society.](/cms/asset/61a01380-6885-47a5-af07-a62813e74e64/gmos_a_1916014_f0005_oc.jpg)
Figure 6. (Colour online) The table at the top shows the respective performance of different descriptors for different algorithms in terms of R, and root mean square error (RMSE). The bottom figure shows the parity plots for ML-predicted methane uptake versus GCMC simulated data on (a) DT, (b) Poisson regression, (c) SVM, and (d) RF models. The colour scale of the right indicates number of times or number of hMOFs that had the equivalent GCMC and ML result [Citation108]. Reprinted with permission from American Chemical Society.
![Figure 6. (Colour online) The table at the top shows the respective performance of different descriptors for different algorithms in terms of R2, and root mean square error (RMSE). The bottom figure shows the parity plots for ML-predicted methane uptake versus GCMC simulated data on (a) DT, (b) Poisson regression, (c) SVM, and (d) RF models. The colour scale of the right indicates number of times or number of hMOFs that had the equivalent GCMC and ML result [Citation108]. Reprinted with permission from American Chemical Society.](/cms/asset/e8f38689-3ade-4d24-b4dd-85bba38b0fcb/gmos_a_1916014_f0006_oc.jpg)
Figure 7. (Colour online) Parity plots for comparing CO selectivity data over N
prediction for different ML methods with respect to GCMC calculations [Citation110]. The correlation coefficient R
and Spearman ranking correlation coefficient (SRCC) results are also shown for each model. Reprinted with permission from American Chemical Society.
![Figure 7. (Colour online) Parity plots for comparing CO2 selectivity data over N2 prediction for different ML methods with respect to GCMC calculations [Citation110]. The correlation coefficient R2 and Spearman ranking correlation coefficient (SRCC) results are also shown for each model. Reprinted with permission from American Chemical Society.](/cms/asset/db22b012-0082-4710-8cea-76c868552119/gmos_a_1916014_f0007_oc.jpg)
Figure 8. (Colour online) Scatter plots for Methane and CO uptake prediction at different pressure based on AR-RDF descriptors versus predicted via GCMC calculations of ∼25000 MOF structures [Citation119]. The correlation coefficient R
is shown for all the cases. All the calculation are done at industrial pressure swing adsorption (PSA) conditions. Reprinted with permission from American Chemical Society.
![Figure 8. (Colour online) Scatter plots for Methane and CO2 uptake prediction at different pressure based on AR-RDF descriptors versus predicted via GCMC calculations of ∼25000 MOF structures [Citation119]. The correlation coefficient R2 is shown for all the cases. All the calculation are done at industrial pressure swing adsorption (PSA) conditions. Reprinted with permission from American Chemical Society.](/cms/asset/64de0f47-bb20-4b9c-9266-180f56d550f6/gmos_a_1916014_f0008_oc.jpg)
Figure 9. (Colour online) The workflow for generating energy descriptor for hydrogen storage [Citation133]. The procedure begins with sampling the potential energy distribution of H-MOF interaction in multiple even spaced grid, followed by binning those energy in a histogram. Each of the bins then form an identifier in the energy descriptor of that MOF represented by the input vector
. Reprinted with permission from American Chemical Society.
![Figure 9. (Colour online) The workflow for generating energy descriptor for hydrogen storage [Citation133]. The procedure begins with sampling the potential energy distribution of H2-MOF interaction in multiple even spaced grid, followed by binning those energy in a histogram. Each of the bins then form an identifier in the energy descriptor of that MOF represented by the input vector Xi. Reprinted with permission from American Chemical Society.](/cms/asset/07580619-4d32-45c2-bace-3f43cd689ad7/gmos_a_1916014_f0009_oc.jpg)
Figure 10. (Colour online) A latent representation of cages, which is simply the rows of the matrix – which is an equivalent form of Equation (Equation6
(6)
(6) )'s right hand side term
– embedded into 2D by t-SNE [Citation134]. The coloured points shows the simulated Xe/Kr selectivity of an isolated cage structure at 298 K in an empty box. Furthermore, the points nearby are nearer to each other in the latent cage space and thus are likely to exhibit similar Xe/Kr selectivity while cages marked ‘X’ have too small a window for xenon to enter into the cavity. Reprinted with permission from ACS (American Chemical Society). Further permissions related to the material excerpted should be directed to the ACS.
![Figure 10. (Colour online) A latent representation of cages, which is simply the rows of the matrix UνΣν – which is an equivalent form of Equation (Equation6(6) RSS=∑i=1n∥Xi−∑j=1kαijZi∥2(6) )'s right hand side term UνΣν≡σiui[k] – embedded into 2D by t-SNE [Citation134]. The coloured points shows the simulated Xe/Kr selectivity of an isolated cage structure at 298 K in an empty box. Furthermore, the points nearby are nearer to each other in the latent cage space and thus are likely to exhibit similar Xe/Kr selectivity while cages marked ‘X’ have too small a window for xenon to enter into the cavity. Reprinted with permission from ACS (American Chemical Society). Further permissions related to the material excerpted should be directed to the ACS.](/cms/asset/2a00d493-5d8c-4a4c-b15b-97cbad1a2600/gmos_a_1916014_f0010_oc.jpg)
Table 3. A list of some more second-order descriptors with applications and material class.