Figures & data
Table 1. Variable ranges and physical parameter settings (in SI units) for the paper helicopter experiment. Flight time is the measured response.
Figure 2. Logistic regression example: (a) Two-dimensional projections of the SIL-optimal, pseudo-Bayesian D-optimal and central composite designs. (b) Boxplots of 20 Monte Carlo approximations of the expected self-information loss [Equation2[2] ] using B = 20, 000 simulations for the SIL-optimal, Pseudo-Bayesian D-optimal and central composite designs.
![Figure 2. Logistic regression example: (a) Two-dimensional projections of the SIL-optimal, pseudo-Bayesian D-optimal and central composite designs. (b) Boxplots of 20 Monte Carlo approximations of the expected self-information loss [Equation2[2] ] using B = 20, 000 simulations for the SIL-optimal, Pseudo-Bayesian D-optimal and central composite designs.](/cms/asset/acc7bb86-12ce-4dd2-8898-cb8f683adabc/lqen_a_1246045_f0002_b.gif)
Figure 3. Logistic regression example: One-dimensional projections of the SIL-optimal, pseudo-Bayesian D-optimal, and central composite designs.
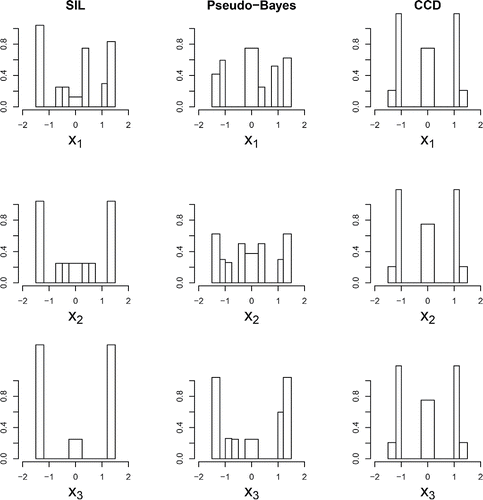
Table 2. Log-linear regression example: Minimally supported Pseudo-Bayesian D-optimal design under uniform prior distribution [Equation8[8] ]; γ = 0.6 for α = 0.5 and γ = 0.455 for α = 0.75.
Table 3. Log-linear regression example: SIL-optimal designs under uniform prior distribution [Equation8[8] ] for (a) α = 0.5 and (b) α = 0.75.
Figure 4. Log-linear regression example: Boxplots of 20 Monte Carlo approximations of the expected self-information loss [Equation2[2] ] using B = 20, 000 simulations for the SIL-optimal and minimally supported Pseudo-Bayesian D-optimal designs (MSPBD) under uniform prior distributions [Equation8
[8] ] for (a) α = 0.5 and (b) α = 0.75.
![Figure 4. Log-linear regression example: Boxplots of 20 Monte Carlo approximations of the expected self-information loss [Equation2[2] ] using B = 20, 000 simulations for the SIL-optimal and minimally supported Pseudo-Bayesian D-optimal designs (MSPBD) under uniform prior distributions [Equation8[8] ] for (a) α = 0.5 and (b) α = 0.75.](/cms/asset/aa636d4c-804b-4740-93bf-f9e0b4df3fe9/lqen_a_1246045_f0004_b.gif)
Table 4. Helicopter experiment: (a) SEL-optimal and (b) V-optimal designs.
Figure 5. Helicopter experiment: Boxplots of 20 Monte Carlo approximations of the average expected posterior variance [Equation3[3] ] from B = 20, 000 simulations.
![Figure 5. Helicopter experiment: Boxplots of 20 Monte Carlo approximations of the average expected posterior variance [Equation3[3] ] from B = 20, 000 simulations.](/cms/asset/b84c9381-25e8-4b74-852f-4b99e37b3d23/lqen_a_1246045_f0005_b.gif)