Figures & data
Table 1. Summary statistics.
Figure 1. Changes in aggregate employment, stock, real rent and vacancy rate. Panel A: Office employment and office floorspace over period 1981–2018. Panel B: Real office rent and office vacancy rate over period 1981–2018.
Note. The graphs are based on aggregate series for the sample of 18 MSAs that have data spanning 1981–2018. Employment and stock were summed across the set of markets, while real rent per square foot per annum and vacancy rate were computed as stock-weighted averages of the series for each MSA in the sample.
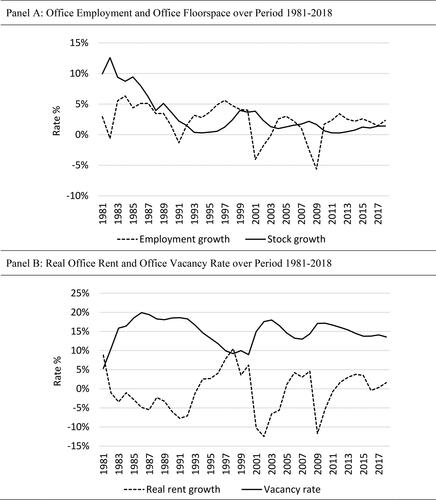
Figure 2. Median vacancy rate and dispersion of vacancy rates across MSAs by year. Panel A: Sample of 18 MSA office markets over period 1980–2018. Panel B: Sample of 61 MSA office markets over period 1990–2018.
Note. The box and whisker plots show the interquartile range (spanned by box) and the full range (from minimum to maximum) in recorded office vacancy rates for that year across the sample of MSAs. The horizontal line inside each box indicates the median vacancy rate in the sample that year.
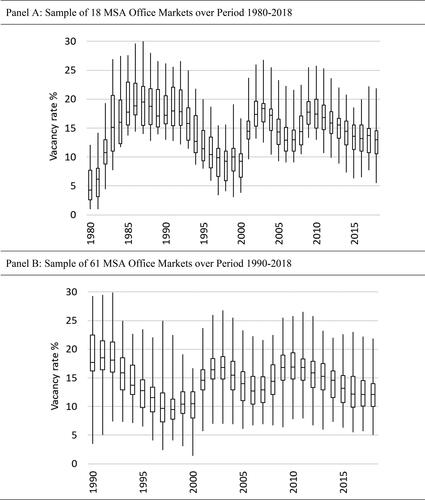
Table 2. Rental change models with a constant NVR.
Figure 3. Estimates of the natural vacancy rate from the short run rent model using rolling 10-year windows. Panel A: Sample of 18 MSAs for windows ending 1990–2018, annual LHS and quarterly RHS. Panel B: Sample of 61 MSAs for windows ending 2000–2018, annual LHS and quarterly RHS.
Note. Model A is the traditional rental adjustment model shown in EquationEquation (6)(6)
(6) . Model B adds the residual error from EquationEquation (7)
(7)
(7) to the specification of Model A. While we also estimated EquationEquation (8)
(8)
(8) , a short run rent model that includes shock variables, estimates of the natural vacancy rate based on this are unstable so are not shown. In each case, the NVR is estimated from the regression coefficients as –β0/β1.
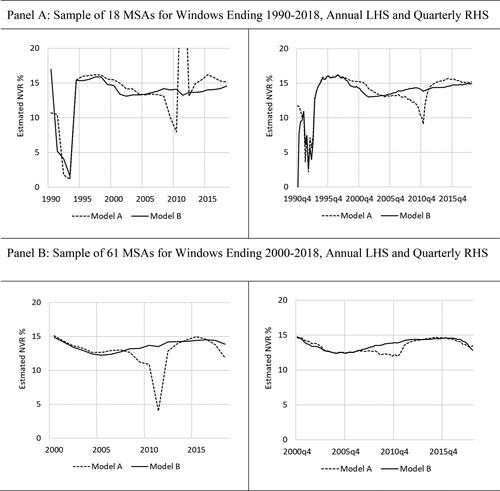
Table 3. Cross-section variation in the NVR from the short run ECM models.
Figure 4. Estimates of the natural vacancy rate based on time fixed effects in a short run rent model, annual data. Panel A: Sample of 18 MSA office markets over period 1980–2018. Panel B: Sample of 61 MSA office markets over period 1990–2018.
Note. Model A is the traditional rental adjustment model shown in EquationEquation (6)(6)
(6) . Model B adds the residual error from EquationEquation (7)
(7)
(7) to the specification of Model A. Model C adds shock variables and is shown in EquationEquation (8)
(8)
(8) . All three models include time and MSA fixed effects. The results from estimation of these models on quarterly frequency data show the same general pattern. Because they are much noisier, they are not shown.
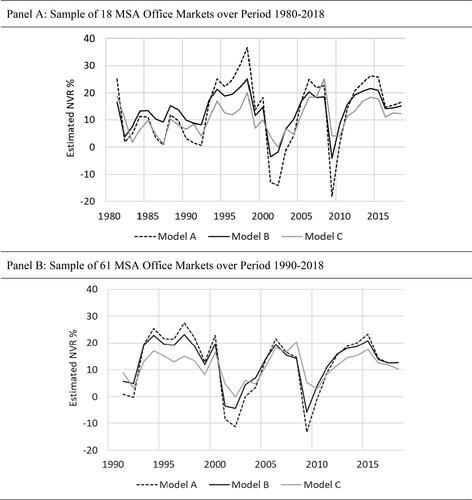
Table 4. Estimates of the NVR from persistence models for whole US.
Table 5. Estimates of the NVR from persistence models using panel models.
Figure 5. Estimates of time variation in natural vacancy rate using persistence models. Panel A: Voith and Crone’s (Citation1988) method applied to annual data, 18 MSAs (LHS) and 61 MSAs (RHS). Panel B: Marston’s (Citation1985) method applied to annual data, 18 MSAs (LHS) and 61 MSAs (RHS). Panel C: Grenadier’s (Citation1995) method applied to annual data, 18 MSAs RHS and 61 MSAs LHS.
Note. The Voith & Crone specification is shown in EquationEquation (15)(15)
(15) , the Marston specification in EquationEquation (12)
(12)
(12) and the Grenadier specification in EquationEquation (16)
(16)
(16) . The results indicate the percentage point variation from the mean natural vacancy rate for each model shown in . The use of an MSA persistence term makes little difference from assuming common persistence across the set of markets, so the lines are very similar. Results from estimation of the models on quarterly frequency data show the same general pattern, although they are much noisier, so are not shown.
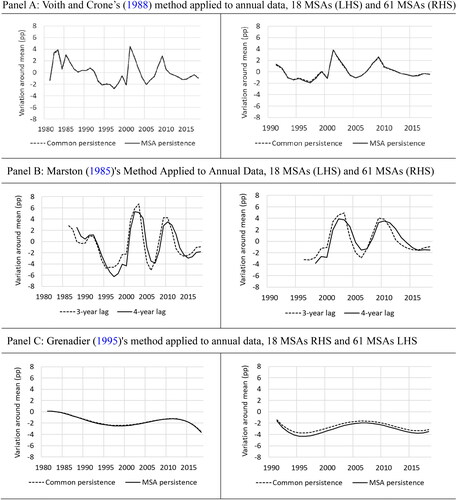