Figures & data
Figure 1. Schematic description of the workflow applied in this study. The training set for the ML algorithms consists of three groups of data: pre-defined process parameters, process signatures extracted from in-situ image data, and target features. The input features were selected based on spearman<apos;>s correlation with target features. Six different ML algorithms were implemented and compared for predictive performance.
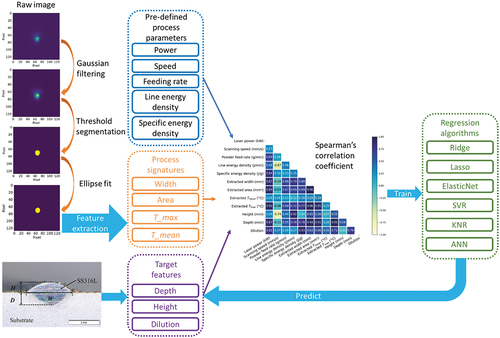
Figure 2. Schematic of the combined experimental apparatus, including laser head, infrared (IR) camera, and laser optics (fibre optic, collimating lens, dichroic mirror, and focusing lens).
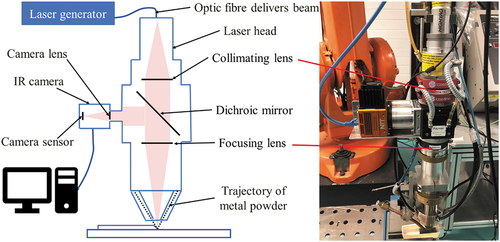
Figure 3. Cross-section of typical single-track deposition (Trial 59). ,
, and
denote track height, depth, and width, respectively.
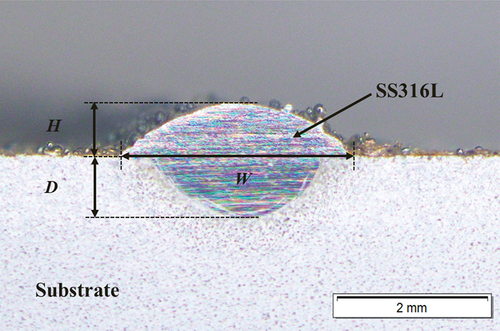
Table 1. Printing parameters used to fabricate all 60 single-track samples. The precisions of the laser power, scanning speed, and powder feed rate was 1 W, 1 mm/s, and 0.1 g/min, respectively
Figure 4. Row width distribution from the threshold segmentation image shown in , column 3, trial 58.
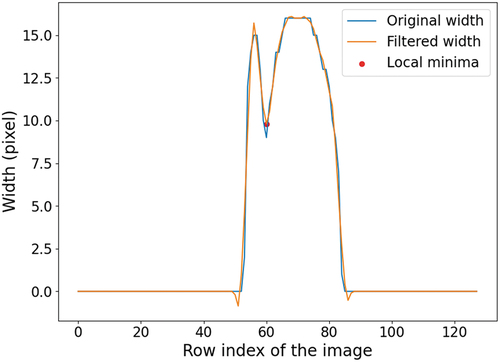
Table 2. Three typical raw melt pool images and their subsequent processing steps
Figure 5. (a) Comparison between the track width measured via an optical microscope and the melt pool width after all the image processing steps described in section 2.3. The (b) absolute errors between two widths.
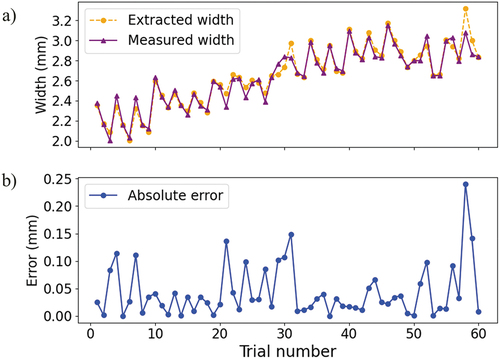
Table 3. Error evaluations between track width measured via an optical microscope and the melt pool width after all the image processing steps described in section 2.3. The precisions of MAE and MXAE are 1 µm
Figure 6. Spearman<apos;>s rank correlation coefficient map for each variable pairing from the predefined process parameters extracted melt pool features and measured track geometries.
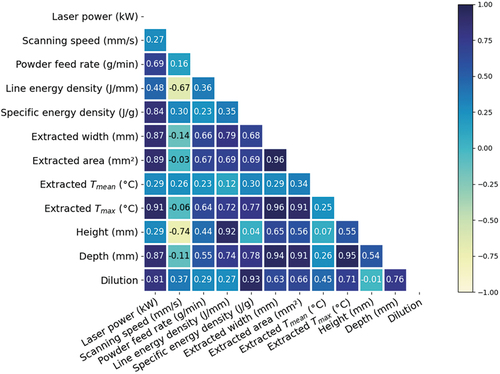
Table 4. Input and output variables for all machine learning models tested here
Table 5. Hyperparameter candidates for different supervised machine learning algorithms used in this research
Table 6. Optimised hyperparameter for all machine learning models tested here
Table 7. R2 values for all machine learning models tested here. These data characterise how well each regression model fits the observed data (measured melt pool depth, height, and dilution) for each train, CV, and test datasets
Table 8. Error measure values when predicting melt pool depth for all machine learning models tested here. The rank assigned to each model is calculated from its mean rank when the models are compared according to their MAE, MXAE, MAPE, and MXAPE values. The precisions of the dimensioned error measures, MAE and MXAE, are both 1 µm
Figure 7. Mean Absolute Error (MAE) and standard deviation for melt pool depth prediction for all machine learning models tested here.
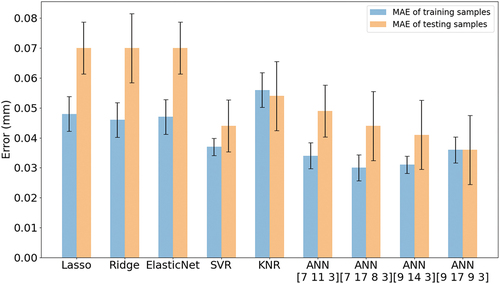
Figure 8. Mean Absolute Error (MAE) and standard deviation for melt pool height prediction for all machine learning models tested here.
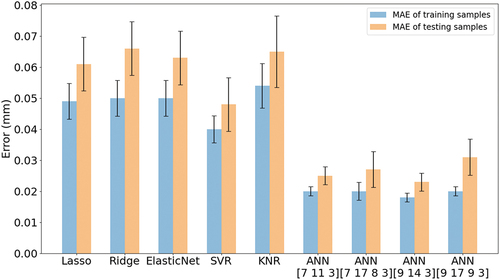
Table 9. Error measure values when predicting melt pool height for all machine learning models tested here. The rank assigned to each model was calculated from its mean rank when the models were compared by their MAE, MXAE, MAPE, and MXAPE values. The precisions of the dimensioned error measures, MAE and MXAE, are 1 µm
Figure 9. Mean Absolute Error (MAE) and standard deviation for melt pool dilution prediction for all machine learning models tested here.
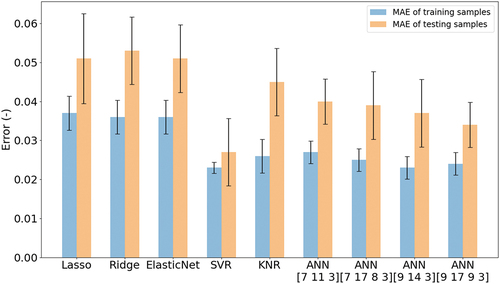
Table 10. Error measure values when predicting melt pool dilution for all machine learning models tested here. The rank assigned to each model is calculated from its mean rank when the models are compared by their MAE, MXAE, MAPE, and MXAPE values
Table 11. Rank values for predicting melt pool depth, height, and dilution for all the machine learning models tested in this investigation. A smaller average rank indicates the better overall performance of the model