Abstract
The paper explores the potential role of Machine learning (ML) in supporting the development of a company’s Performance Management System (PMS). In more details, it investigates the capability of ML to moderate the complexity related to the identification of the business value drivers (methodological complexity) and the related measures (analytical complexity). A second objective is the analysis of the main issues arising in applying ML to performance management. The research, developed through an action research design, shows that ML can moderate complexity by (1) reducing the subjectivity in the identification of the business value drivers; (2) accounting for cause-effect relationships between business value drivers and performance; (3) balancing managerial interpretability vs. predictivity of the approach. It also shows that the realisation of such benefits requires a combined understanding of the ML techniques and of the performance management model of the company to frame and validate the algorithm in light of the context in which the organisation operates. The paper contributes to the literature analysing the role of business analytics in the field of performance management and it provides new insights into the potential benefits of introducing an ML-based PMS and the issues to consider to increase its effectiveness.
Notes
1 Okwir et al. (Citation2018) framework identifies a third source of complexity namely technological complexity. However, it is not specifically linked to the identification and measurement of business value drivers but to the role of IT systems and platforms in supporting PMSs (Nudurupati et al. Citation2011, Nudurupati and Bititci Citation2005).
2 A second less relevant intervention was that from the variable “Amount recovery plan” we derived a new binary variable “Recovery plan agreed” equal to 1 when the amount was positive and equal to 0 when the amount was 0.
3 The higher the decrease of the index when the variable is included, the higher the impact of the independent variable on the dependent one.
4 At the end of the selection process the following independent variables were considered: “Procedure Outcome” as the dependent variable; “Recovery Plan Agreed”, “Amount outstanding”, “Operator. B”, “Year of birth. B”, “Solvent”, “E-mail received”, “Macroregion”, “Sex” as independent variables.
5 We used a K-fold validation with K = 5. The general K-Fold algorithm shuffles the dataset randomly and splits it into k groups. Then, for each unique group it takes the group as a test dataset and takes the remaining groups as a training dataset. Then, it fits a model to the training set and evaluates it on the test set, then it retains the evaluation score (the performance measures specifically used will be discussed later in this section). Finally, it summarises the skill of the model using the mean of the performance scores achieved by each fold.
6 AUC measures the extent to which a model is capable of distinguishing between classes. The higher the AUC, the better the model is at predicting each class. An excellent model has an AUC close to 1, which means it has a good measure of separability. When AUC is equal to 0.5, it means that the model is not able to achieve any class separability. If AUC = 0 the model provides completely wrong predictions, predicting negative outcomes as positive and vice versa.
Additional information
Notes on contributors
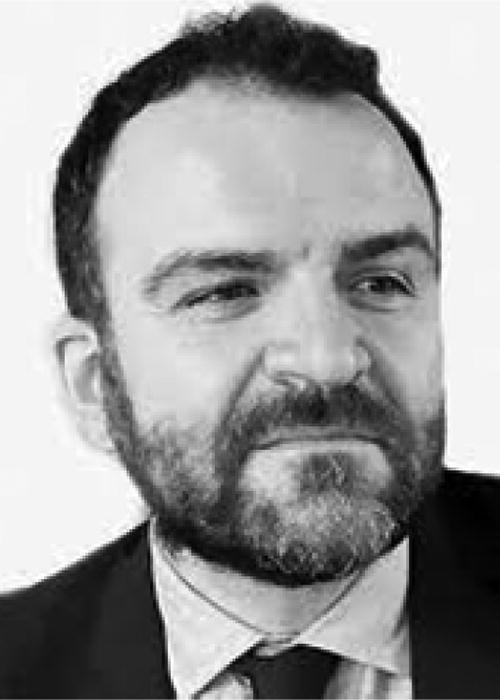
Franco Visani
Franco Visani, PhD, is Associate Professor of Management Accounting at the University of Bologna. He is part of the Faculty of several MBA and Executive MBA programs of Bologna Business School (University of Bologna), where he is also the director of the Hybrid MBA. He is actually involved in a series of international researches focused on the evolution of management accounting and specifically on adoption of mathematical and statistical approaches to strategic and operational control. His other research interests are strategic cost management and supply chain cost management and control.
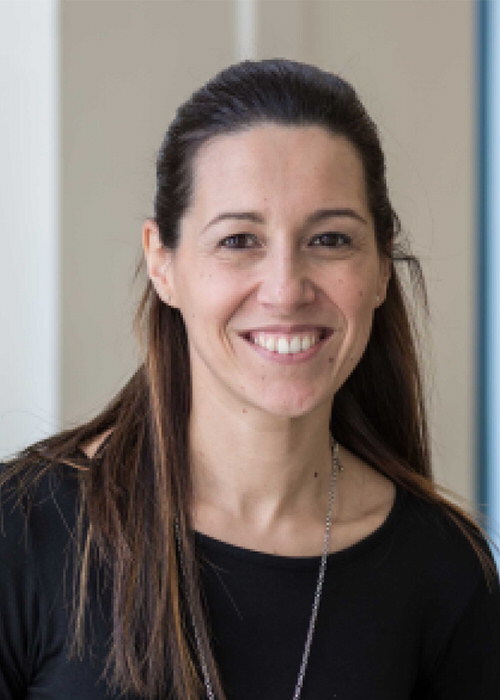
Anna Raffoni
Anna Raffoni, PhD, is Associate Professor in Accounting and Finance at Loughborough University School of Business and Economics. She previously held academic positions at University of East Anglia and Cranfield University School of Management. Anna has been involved in a number of national and international research projects and published several articles. Her research interests are analytics performance management, accounting and digitalisation, performance management and servitization.
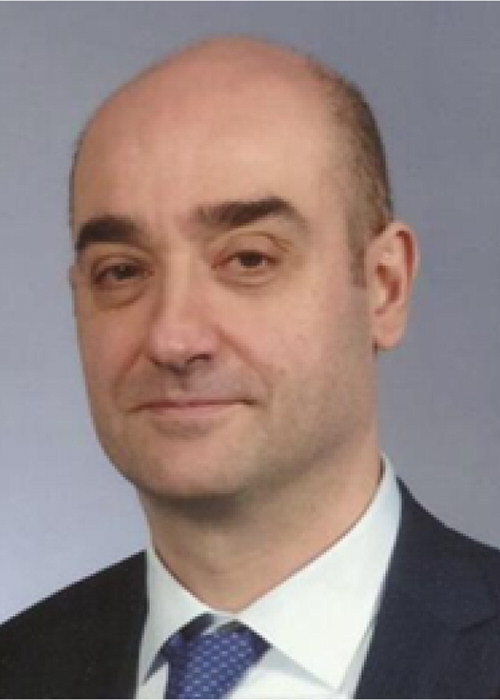
Emanuele Costa
Emanuele Costa is a PhD. Student at the University of Cardiff (UK). Emanuele educational background includes a BSc. in Mathematics and Computer Science from Lancaster University (UK) and a MSc. in Artificial Intelligence from Edinburgh University (UK) and an Executive MBA at the University of Bologna (Italy). He is also a certified Wealth Manager (CISI) and has several years of experience in the Financial Industry, working for major investment banks like Barclays, UBS and Goldman Sachs.