Figures & data
Table 1. Sample input vectors representing semantic co-occurrence.
Figure 1. A subset of activation maps after training for Simulations 1A and 1B. (Top) Where Layer 1 acquires unique sensorimotor representations for each concept, Layer 2 acquires representations of semantic category (object, or action). (Bottom) Adjusting the semantic similarity scaling parameter τ allows finer semantic categories to emerge. Here, in Layer 3, separate categories for “NOUN_FOOD” (LOLLIPOP, POTATOCHIP, GUM), “NOUN_AGENT” (OWL, DADDY, MOMMY), and “NOUN_OBJECT” (TRICYCLE, BUCKET) are evident.
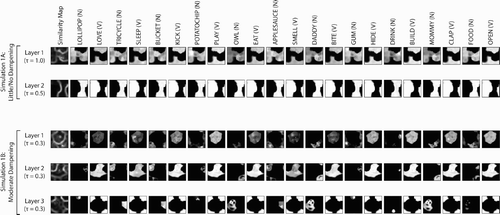
Figure 2. The network architecture used in Simulation 2. Layers 1 and 2 represent the subset that is identical to Simulation 1, where a recurrent grammar-learning network has been appended to acquire valid sequences of parts of speech distilled from Layer 2.
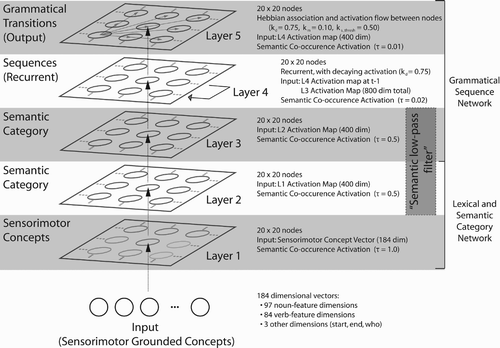
Table 2. Example sentences used in both training (top) and test (bottom) sets, across simple, right-branching, and centre-embedded sentence structures.
Figure 3. The results of Simulation 2 (sensorimotor input set) for a novel centre-embedded sentence, “NAPKIN – WHO – SUN – BRING – WISH – APPLE”. Here, the similarity map represents a scaled relative difference in the Euclidian distance of the weight vectors contained in nearby nodes, out to some small radius (where black represents very similar representations in adjacent nodes, and white represents dissimilar adjacent nodes). The input layer (Layer 1) represents sensorimotor conceptual information, where layers 2 and 3 represent semantic category. The output layer (Layer 5) generates unique grammatical predictions for each transition. The activation patterns in Layers 2–5 are nearly identical to those in the case of any given familiar centre-embedded sentence, indicative of a network that is strongly systematic. (Note that this example simulation was run at a network size of 32×32 for all layers to generate higher resolution images for visualisation.)
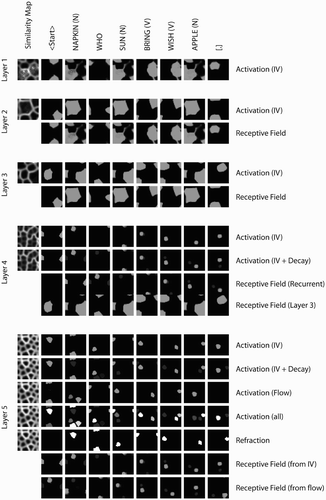
Figure 4. Example tagged activation map keys automatically generated from the activation patterns of one sensorimotor and one localist training run. Clusters representing the transitions of a finite-state automation representing the Van der Velde et al. grammar are clearly evident in the sensorimotor grounded map, with (for example) two clusters representing the two possible transitions to the “Who” marker, three clusters representing the three possible transitions to an “End” marker, four transitions to a “Verb”, and five transitions to a “Noun”. Topographic organisation in the localist key is less clear, and some nodes display involvement for multiple transitions.
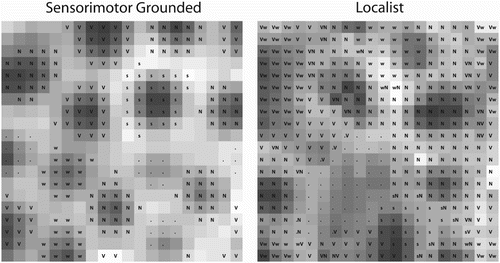
Figure 5. Mean and best-average GPE for each transition in the van der Velde et al.’s grammar for both the sensorimotor grounded and localist test sets of Simulation 2, averaged across 10 simulations. Replacing the sensorimotor grounded sensorimotor input set with ungrounded representations greatly increases GPE, particularly for deep transitions in the right-branching and centre-embedded structures.
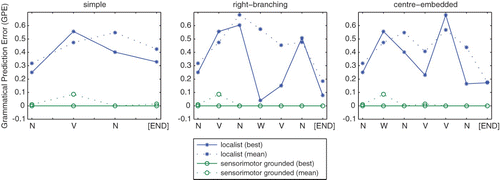
Figure 6. Mean transitional prediction values across each possible transition in the Van der Velde et al. grammar averaged across 10 simulations using the sensorimotor grounded input set. The network displays excellent performance across all transitions. Ungrammatical transitions are marked with an asterisk.
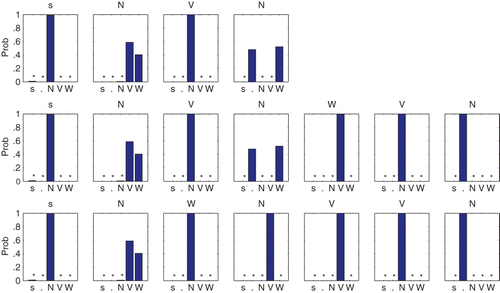
Table 3. Mean GPE for Simulation 2 across simple, right-branching, and centre-embedded sentence structures for both sensorimotor grounded and localist input sets. Values in parentheses represent the mean of the standard deviations across all transitions in a given set.
Figure 7. Exemplary activation inthe output layer of the Chimaera model (Layer 5) after training for simple, right-branching, and centre-embedded sentence structures on sensorimotor input. Predictive flow maps are also included, showing the Chimaera's prediction mechanism successfully flowing activation to the next grammatical transition in each sentence structure. Here, all maps are scaled to activation values between 0 (white) and 1 (black).
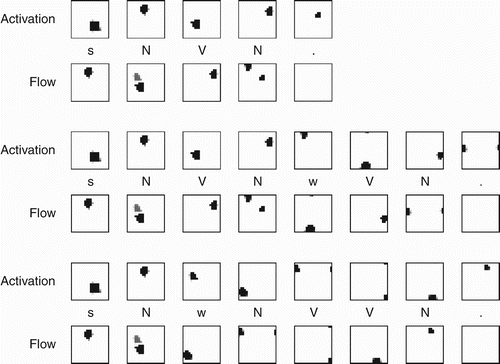
Figure 8. Histogram of proportions of abstraction percentages across simple, right-branching, and centre-embedded sentences for sensorimotor grounded and ungrounded localist input sets. Histogram bins represent trials with abstraction percentages equal to or less than the label value, but greater than the next smallest bin label. Proportions add to 1.0 for each of the four datasets, with the exception of the localist test set, where failed response trials are not included in any bin. Values towards 0% signify that the network has represented a given transition in some sentence type using different activation maps, and is favouring an instance-based representation scheme (i.e. a given sentence structure produces different patterns of activation in the output layer when different words are used in that sentence structure). Conversely, values towards 100% signify that the network is representing a given transition in some sentence structure using the same activation map, regardless of the specific words used in that sentence. Abstraction percentages near 100% are indicative of strongly systematic behaviour. The sensorimotor grounded dataset displays strong systematicity, where the ungrounded localist dataset includes a mix of values between strongly systematic and instance-based.
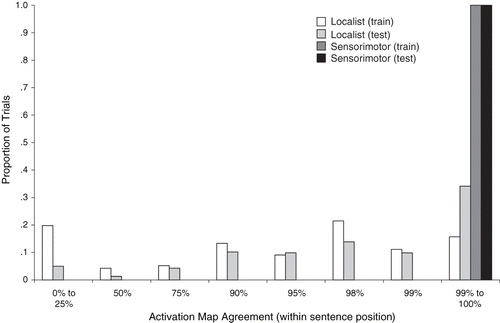