Figures & data
Figure 1. This figure shows an example three-agent DCEE. Each agent controls one variable and the settings of these three variables determine the reward of the two constraints (and thus the total team reward).
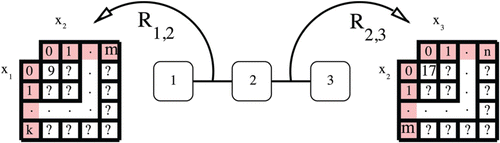
Figure 3. The signal scheme index for each DCEE agent, and its corresponding (greenoffset, greentime) value, defining an active phase of 60 s. Note that greenoffset and greentime increase at 5-s intervals, a necessary discretisation.
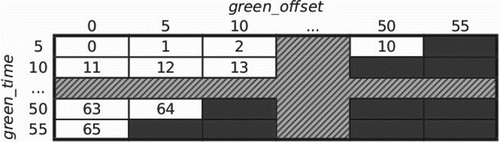
Figure 4. The full signal scheme for an intersection, given a specific active phase. Time flows from left to right: the calculated active phase is active for North–South in the first 60 s, before switching to East–West in the next 60 s. The whole signal scheme repeats after 120 s total.

Figure 5. Average delay and throughput for a light traffic level (10 cars spawned per minute at each entrance). Error bars show one standard deviation.
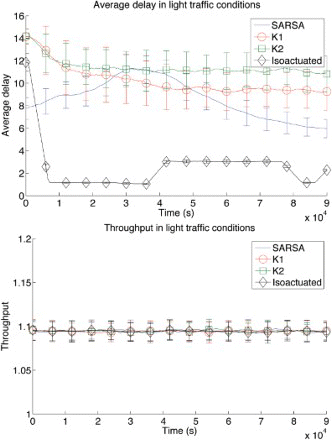
Figure 6. Average delay and throughput for a heavy traffic level (30 cars spawned per minute at each entrance). Error bars show one standard deviation.
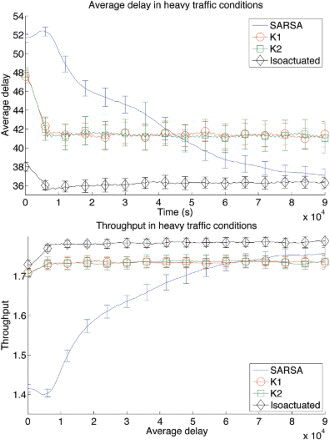
Figure 7. The reward samples observed during a single run with a heavy traffic level and either delay (a) or throughput (b) as a reward signal. Colour indicates the timing of the sample, with blue early in the run, and red at the end of the run. The objectives (minimising delay on x-axis and maximising throughput on y-axis) are observed to be correlated.
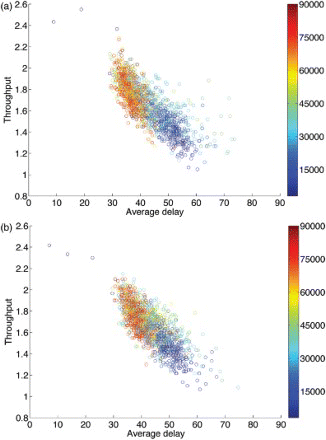
Figure 8. Average delay for a light traffic level (10 cars spawned per minute at each entrance). Comparison of two single-objective approaches and linear scalarisation. Error bars show one standard deviation.
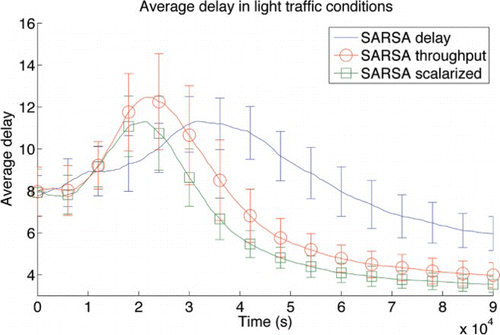
Figure 9. Average delay and throughput for a heavy traffic level (30 cars spawned per minute at each entrance). Comparison of two single-objective approaches and linear scalarisation. Error bars show one standard deviation.
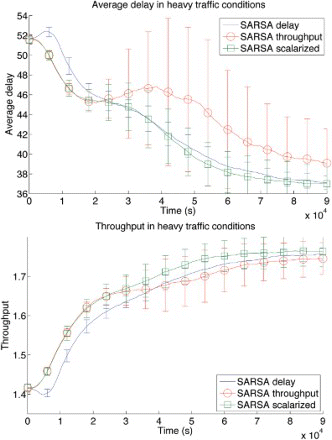
Figure 10. Average delay for a light traffic level (10 cars spawned per minute at each entrance). Comparison of delay, scalarised (delay and throughput), delay-squared and a different scalarised (delay squared and throughput) reward signal. Error bars show one standard deviation.
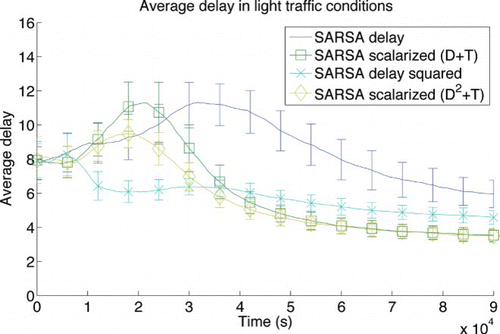
Figure 11. Average delay and throughput for a heavy traffic level (30 cars spawned per minute at each entrance). Comparison of delay, scalarised (delay and throughput) and delay-squared reward signals. Scalarised with delay-squared and throughput yields the same performance as delay-squared alone. Error bars show one standard deviation.
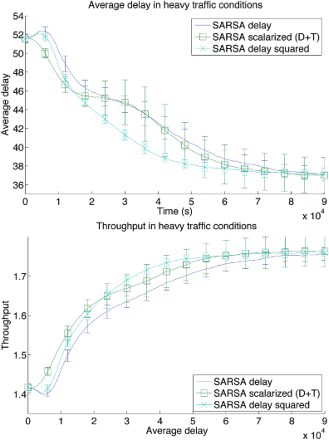