Figures & data
Figure 1. The overall MTRNN architecture with exemplary three horizontally parallel layers: input-output (IO), context-fast (Cf), and context-slow (Cs), with increasing timescale τ, where the Cs layer includes some context-controlling (Csc) units. While the IO layer processes dynamic patterns over time, the Csc units at first time step (t=0) contain the context of the sequence, where a certain concept can trigger the generation of the sequence.
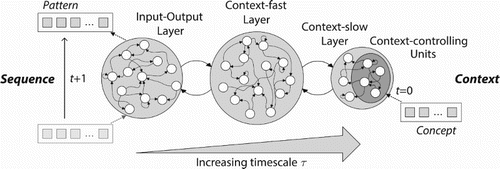
Figure 2. The MTRNN with context abstraction architecture providing exemplary three horizontally parallel layers: context-slow (Cs), context-fast (Cf), and input-output (IO), with increasing timescale τ, where the Cs layer includes some context-controlling (Csc) units. While the IO layer processes dynamic patterns over time, the Csc units abstract the context of the sequence at last time step (t=T). The crucial difference to the MTRNN with context bias is an inversion of the direction of procession and an accumulation of abstract context instead of production from a given abstract context.
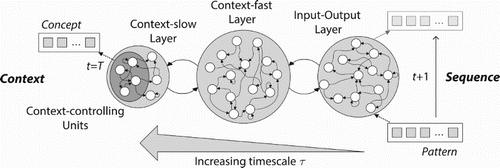
Figure 3. Effect of the self-organisation forcing mechanism on the development of distinct concept patterns for different sequences of contrary cosine waves: training effort (a) and mean and
with standard error bars over varied ψ (b), each over 100 runs; representative developed Csc patterns (c–f) for different sequences for selected parameter settings of no, small, “good”, and large self-organisation forcing, respectively.
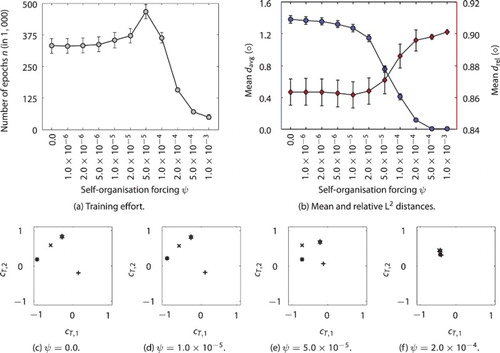
Figure 4. Architecture of the multi-modal MTRNN model, consisting of an MTRNN with context bias for auditory, two MTRNNs with context abstraction for somatosensory as well as visual information processing, and CAs for representing and processing the concepts. A sequence of phonemes (utterance) is produced over time, based on sequences of embodied multi-modal perception.
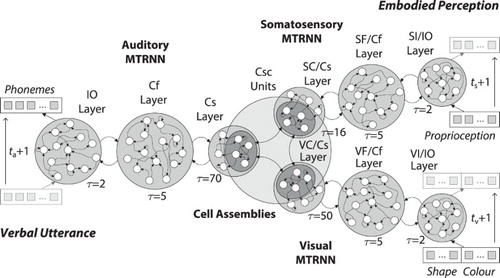
Figure 6. Schematic process of utterance encoding. The input is a symbolic sentence, while the output is the neural activity over neurons times
time steps.
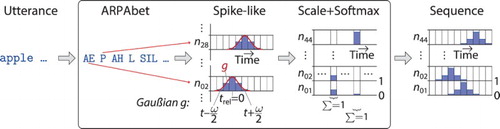
Figure 7. Schematic process of visual perception and encoding. The input is a single frame taken by the NAO camera, while the output is the neural activity over N neurons, with N being the sum over .
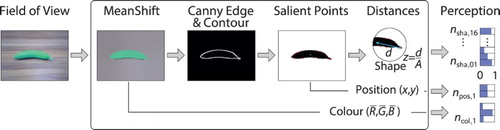
Table 1. Standard meta and training parameter settings for evaluation.
Figure 9. Effect of the self-organisation forcing mechanism on the development concept patterns in the MultiMTRNNs model: mean mixed -score (a) and mixed edit distance (b) – “mixed” measures indicate a combination of training and test results with equal weight, mean of average and relative pattern distances (c), and intra- and intra-cluster distances (d) with interval of the standard error, each over 100 runs and over varied
, respectively; representative developed Csc patterns (e–g) reduced from
to two dimensions (PC1 and PC2) for selected parameter settings of no, “good”, and large self-organisation forcing, respectively. Different words for shapes and colours are shown with different coloured markers (black depicts “position” utterance).
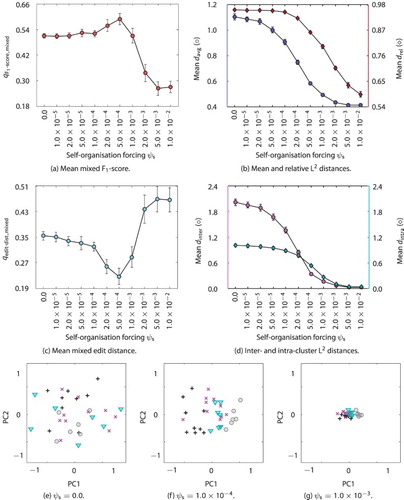
Figure 10. Activity in the Csc units after the model has been activated by proprioception and visual perception for the final internal states (somatosensory and visual) and the initial internal states (auditory), reduced from to two dimensions (PC1 and PC2) and normalised, each. Visualisation a, c, e are shown for an representative example for low and b, d, f for high generalisation.
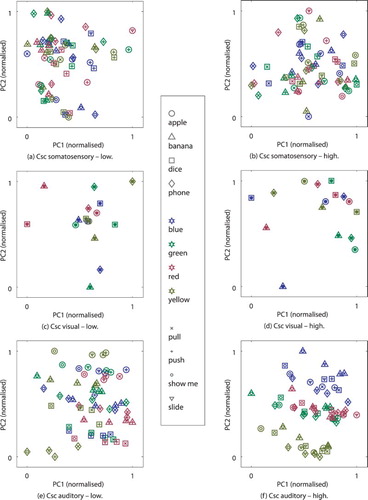