Figures & data
Figure 1. Example images captured from various weathers in CARLA. The quality and clarity of these images are different, thus influencing the performance of the driving models. (a) clear noon, (b) clear noon after rain, (c) heavy rain noon, (d) clear sunset, (e) after rain sunset, (f) soft rain sunset.
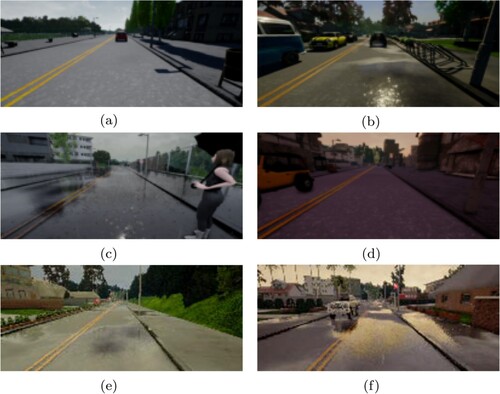
Table 1. The driving results of the CILRS (Codevilla et al., Citation2019) agent in different weather conditions.
Figure 2. The mapping network. The output style codes i.e. clear noon, clear noon after rain, heavy rain noon, and clear sunset, are the input of the generator.
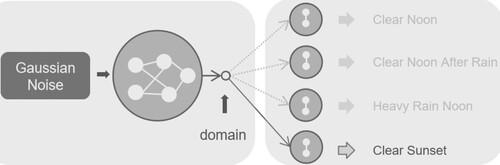
Figure 4. The workflow of Star-CILRS. The proposed method contains two main models: the translation network and the driving model. The translation network takes a source image as input and outputs a generated image with a specific weather style. Given the generated image, the driving model takes actions for autonomous driving.
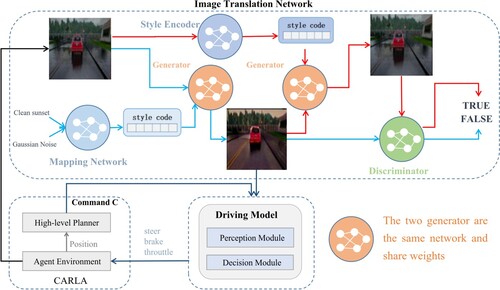
Figure 5. The inference stage of our method. The generator takes a source image and a target style code to output the target image with a specific style. Then, the translated image is fed to the driving model to control the agent.
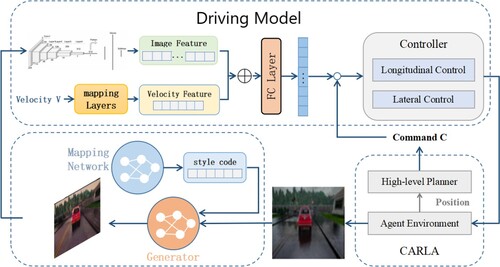
Figure 6. Town01 and Town02 in CARLA (Dosovitskiy et al., Citation2017).
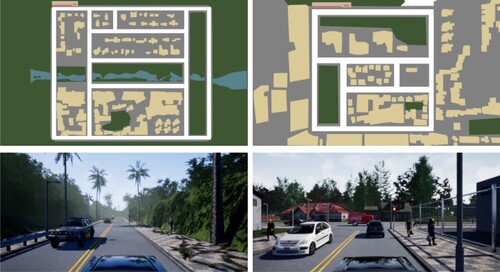
Figure 7. Example images generated by the translation network in empty scenarios. Source images are in the first row and reference images are in the first column where all images are real images. The rest images in the middle are the generated images with the style extracted from the images in the first column. These images are collected in simple task scenarios with only a few vehicles.
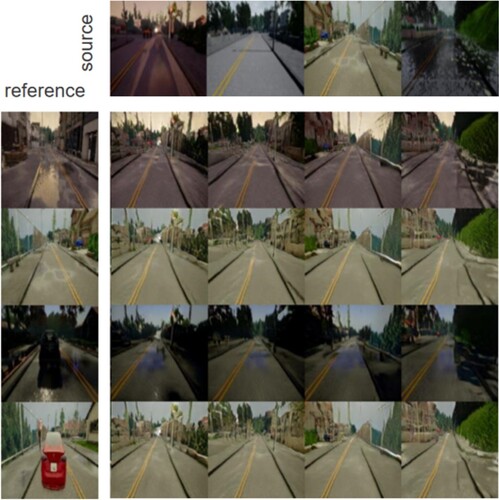
Figure 8. Example images generated with vehicles. Source images with vehicles are in the first row, and reference images are in the first column. The rest of the images in the middle are the generated images.
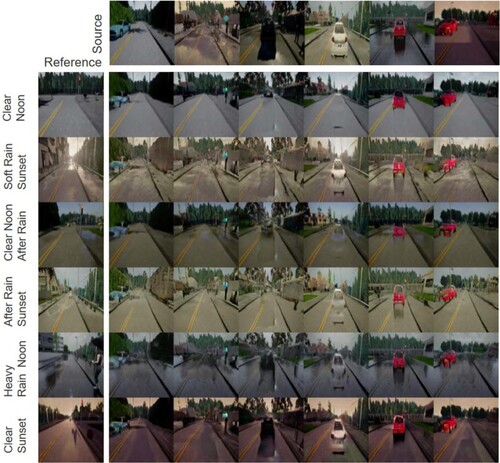
Table 2. Comparison with the state-of-the-arts on the CARLA benchmark in Town02 with training weathers.
Table 3. Comparison with the state-of-the-art on the CARLA benchmark in Town01 with testing weathers.
Table 4. Comparison with the state-of-the-art on the CARLA benchmark in Town02 with testing weathers.