Figures & data
Table 1. Malay NER datasets.
Table 2. Data source.
Figure 1. Dataset construction process. It consists of two parts: preliminary construction and iterative optimisation.
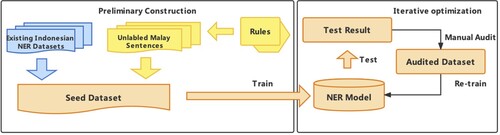
Table 3. Audit guideline.
Table 4. An example of MS-NER.
Figure 2. The MTBR framework structure. Due to space limitation, [B-P, I-P, B-L, I-L, B-O, I-O, O] in the figure represents [B-PER, I-PER, B-LOC, I-LOC, B-ORG, I-ORG, OTHER].
![Figure 2. The MTBR framework structure. Due to space limitation, [B-P, I-P, B-L, I-L, B-O, I-O, O] in the figure represents [B-PER, I-PER, B-LOC, I-LOC, B-ORG, I-ORG, OTHER].](/cms/asset/ed89c314-362a-47f7-b2f4-e3ca6ccd219e/ccos_a_2159014_f0002_oc.jpg)
Figure 3. Probability alignment. If is the first token of the detected entity, the probabilities would flow towards the black arrow; otherwise they would flow towards the red arrow.

Table 5. Model settings.
Table 6. Main performance.
Table 7. Performance of different modules.
Table 8. BRE analysis.
Table 9. Case study. MTBR gives all correct predictions in these cases.
Table 10. MTBR performance on more languages.