Figures & data
Table 1. An dialogue example with different user goals and the evaluation results.
Table 2. Summarisation of related work.
Figure 2. Architecture of the proposed scheduled knowledge distillation for dialogue policy learning.
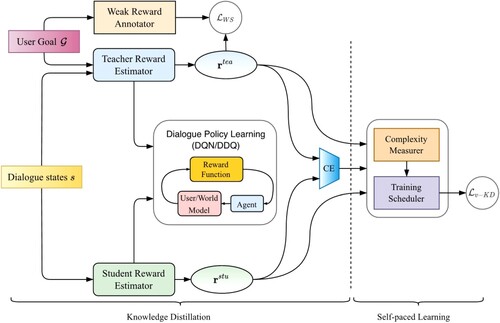
Figure 3. Architecture of teacher and student reward estimators. (a) Teacher reward estimator (b) Student reward estimator.
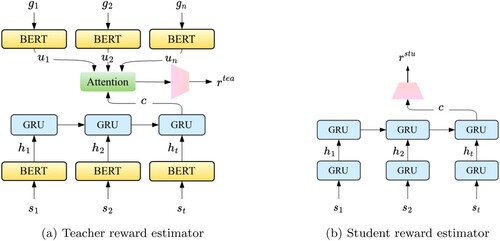
Figure 4. Dialogue state and user goal representations. (a) Multi-hot state representations (b) Embedded state representations (c) Embedded user goal representations.
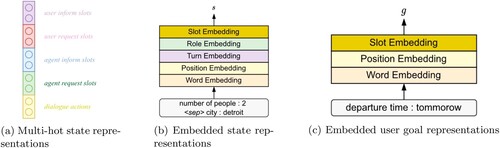
Table 3. The statistics of datasets.
Table 4. Experimental results on Microsoft Dialogue Challenge dataset.
Table 5. Experimental results on MultiWOZ dataset.
Figure 5. Learning curves of agents discarding different components on Movie Ticket Booking dataset. (a) DQN-based agents (b) DDQ-based agents.
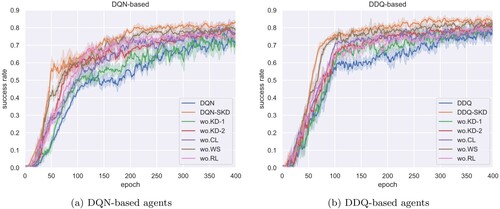
Table 6. Success rate of removing the curriculum learning (CL), knowledge distillation (KD), weak supervision (WS) and reinforcement learning (RL) on Movie-Ticket Booking dataset.
Figure 6. Box plots of DQN and DDQ based agents on Movie-Ticket Booking dataset. The center, bottom and top line of the box show the median, and
percentiles, respectively. The lines that extend from the box represent the expected variation of the data. The shorter the box, the more stable it is. (a) DQN-based agents (b) DDQ-based agents.
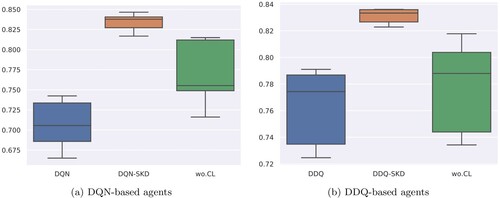
Figure 7. Learning curves of DQN-SKD and DDQ-SKD with different anchor reward . (a) DQN-based (b) DDQ-based.
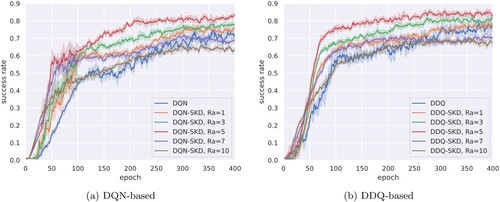
Figure 8. Reward visualisation of a dialogue simulation between a rule based agent and a user simulator. The reward is provided by a trained student reward estimator.
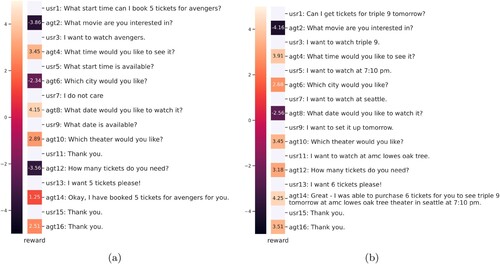
Figure 9. Success rate of different agents dealing with shifty user goals on MultiWOZ. The number in each bar represents success rate degradation compared to that in Table at Epoch=300.
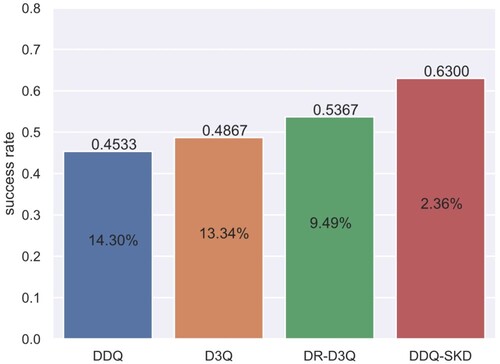
Table 7. Experimental results of DDQ-SKD with different complexity measurer on MultiWOZ dataset.
Table 8. Dialogue examples of simulated user interacting with different agents trained at 100 epochs.
Figure 10. Human evaluation results of DQN, DQN-SKD, DDQ and DDQ-SKD agents. The number of test dialogues are presented in on each bar.
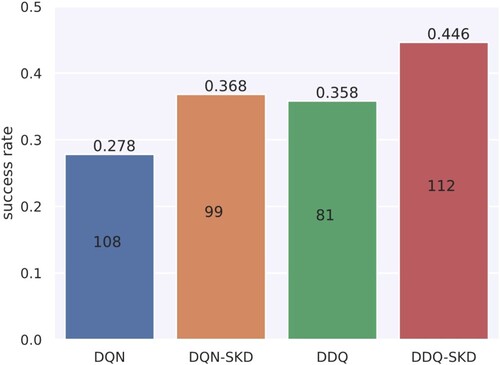
Data availability
The Microsoft Dialogue Challenge and MultiWOZ dialogue datasets analysed during the current study are available in E2EDialog and ConvLab-2 repositories, respectively.