Figures & data
Table 1. List of acronyms.
Table 2. Comparative study of related works.
Table 3. Recent challenges and proposed solutions.
Table 4. Neural network layers.
Table 5. Demonstration of deep neural network layers.
Table 6. Dataset information.
Table 7. Comparison of various feature learning process for application identification.
Table 8. Comparison of SVM and proposed work (NN + SVM) for classification WhatsApp media content (image/text).
Table 9. Comparison of various machine learning models for application identification.
Figure 6. Precision, recall, F1 comparison between models for classifying WhatsApp application from others.
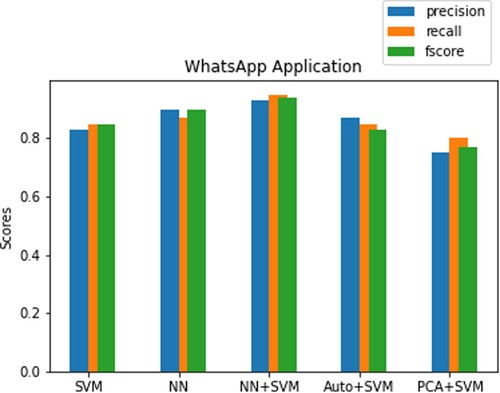
Figure 7. Precision, recall, F1 comparison between models for classifying other application from others.
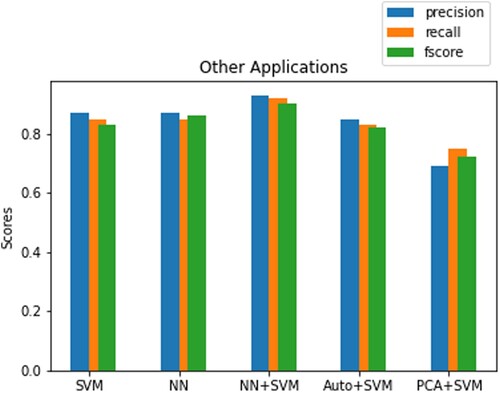
Table 10. Comparison of various machine learning models for classification of WhatsApp media Content (image/text).
Table 11. Comparison of proposed methodology with other state of the art deep learning methods in classifying VPN traffic application.
Table 12. Autoencoder layer details.