Figures & data
Figure 4. DO-Conv process. The calculation method of figure (a) is called feature composition, and the calculation method of the figure (b) is called kernel composition.
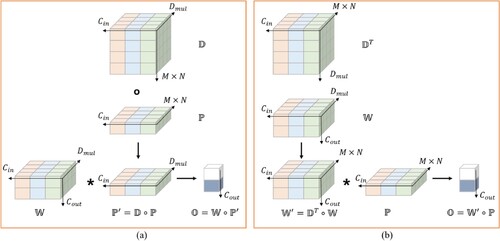
Figure 5. Over-parameterised convolution module. The structure of this module for frequency and spatial feature learning.
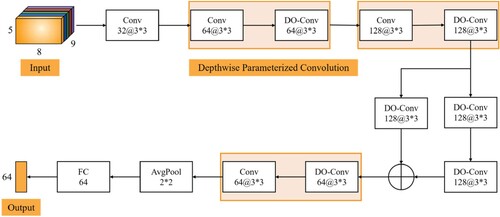
Table 1. Network parameter selection.
Table 2. Ablation studies of each module.
Table 3. Performance comparison of the models on the SEED dataset.
Table 4. Performance comparison of the models on the SEED-IV dataset.