Figures & data
Table 1. Scene categories from the Places205 project (Zhou et al. Citation2014).
Table 2. Scene attributes from the Places205 project (Zhou et al. Citation2014).
Table 3. Rules used to assist in the classification of the photographs as useful, from Antoniou et al. (Citation2016).
Table 4. UA classes of levels 2 and 4 (European Union, 2011, https://cws-download.eea.europa.eu/local/ua2006/Urban_Atlas_2006_mapping_guide_v2_final.pdf).
Figure 1. UA classes for the Paris study region, with locations of all photographs overlaid. The abbreviation “S.L” in the legend refers to the proportion of sealed surface which helps to define that class.
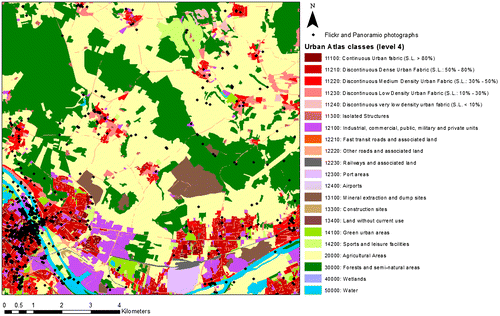
Table 5. Frequency of UA level 2 classes in the data set at the specific location of each photo (point) and in the area around it (buffer).
Table 6. Frequency of UA level 4 classes in the data set at the specific location of each photo (point) and in the area around it (buffer).
Table 7. Confusion matrix, precision, recall and F1-score produced on a stratified test set, with number of photographs equal to 25% of all photographs in the Paris data set (the remainder were used for training the model) for the DT approach.
Table 8. Average precision, recall, and F1-score for the DT approach.
Table 9. Confusion matrix, and the precision, recall, and F1-score for the “usefulness” prediction with the DT approach.
Table 10. Confusion matrix, and the precision, recall, and F1-score for the ‘usefulness’ prediction with the DT approach by tuning the weights such that we limit the number of false positives (increasing precision at the expense of recall).
Table 11. Confusion matrix, precision, recall and F1-score for the “usefulness” prediction with the UW approach.
Table 12. Confusion matrix for UA level 2 and the class prediction based on the scene attributes.
Table 13. Prediction accuracy of land cover with the DT approach on the extraction of UA classes from photographs, considering level 2 classes (7 classes) and level 4 classes (14 classes). “Chance of getting the result at random” is simply the chance of randomly guessing the class, 1/7th and 1/14th respectively.
Table 14. Average unweighted and weighted average of the precision, recall, and F1-score for the level 2 UA classes presented in Table 11.
Figure 4. The example of urban photographs whose geolocation indicates objects which is not the objects of interest.
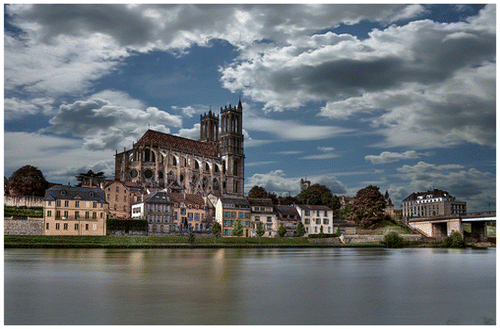