Figures & data
Figure 1. Imagined examples of trajectory polyline intersections with the example of (a) a detected road intersection with traffic lights, and of (b) elevated intersections, in comparison with (c) a trajectory polyline intersection patterns from real taxi trajectory data.
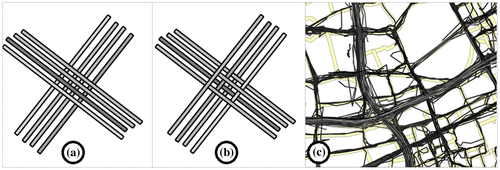
Table 1. Data structure of the inspected taxi FCD set of Shanghai.
Figure 3. Different visual patterns of taxi trajectory polylines at selected infrastructure elements, such as (a) crossroads, (b) crossing with changes in elevation, (c) on-ramps, (d) elevated highway, (e) elevated highway, and, (f) Nanpu bridge on-ramp.
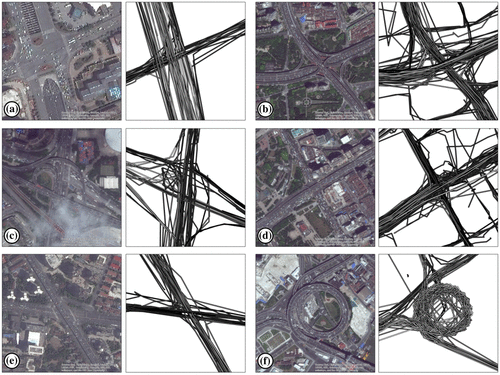
Figure 4. Attribute computation for trajectory polylines with (a) travel time based on average velocity differences, (b) travel time based on distances, and (c) travel time as averaged time stamps.
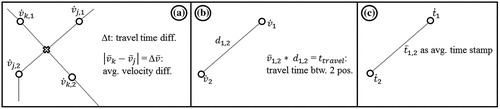
Figure 5. Visualization of (a) used taxi trajectory polylines, (b) resulting TIP extracts, (c) trajectory polylines with self-intersections, and (d) resulting self-intersection point extracts.
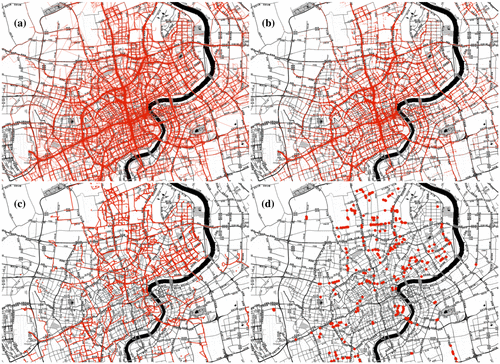
Figure 7. Comparison between the three quantities taxi density, avg. speed, and traffic congestion value c, respectively, for two selected time windows.
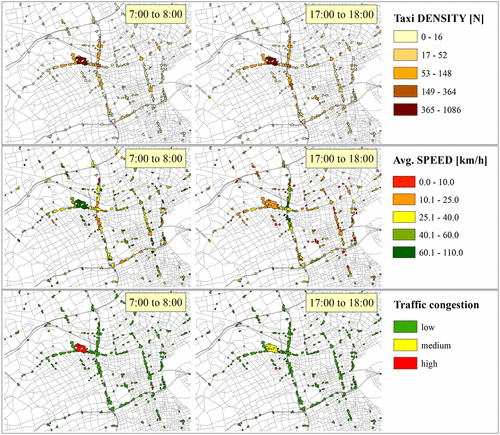
Figure 8. Level of complexity of transportation infrastructure elements for the center area of Shanghai, with (a) counted road network nodes and (b) calculated node density.
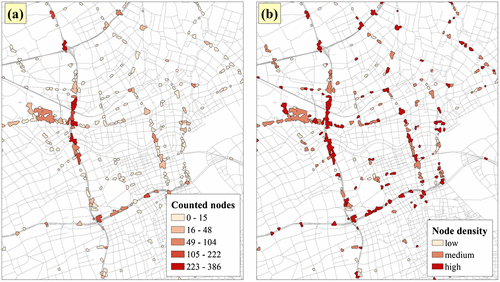
Figure 9. Selection of TIP polygon in the north of Shanghai based on taxi FCD from 8:00 to 9:00 am on 22 February 2007.
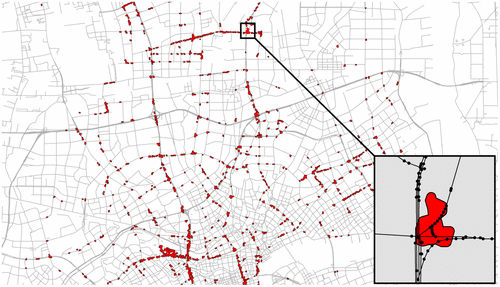