Figures & data
Figure 2. Experiment results on Pavia University data (a) Original Pavia University image. (b) Ground reference map containing nine land-cover classes. (c) Original K-means clustering result (same-colored region belongs to the same subregion). (d) The result of the image segmentation using the OK-means clustering method (each closed region is a subregion). The results of the ML classification after using different dimensionality reduction methods (number of features = 8), (e) OMNF method, (f) KMNF method, (g) OKMNF method, and (h) KM-KMNF method.
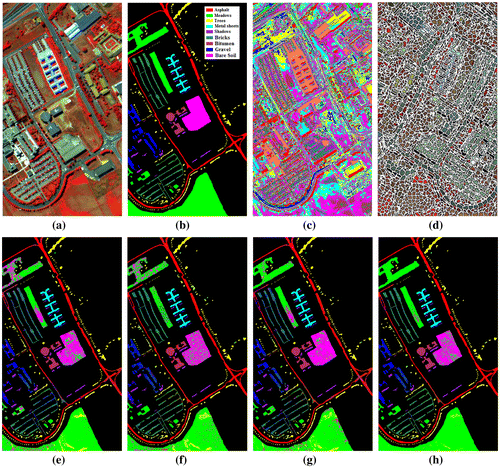
Table 1. Pavia University samples used for training and testing.
Table 2. Overall accuracy of the ML classification on Pavia University image using different dimensionality reduction methods.
Figure 3. Comparison of the accuracies of the ML classification on Pavia University image using different number of features.
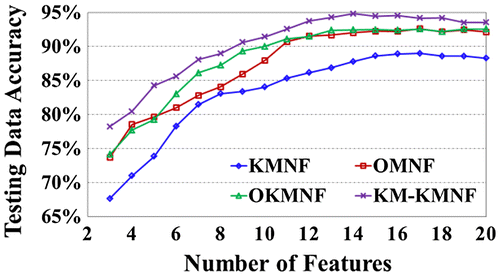
Figure 4. Experiment results on Indian Pines data (a) Original Indian Pines image. (b) Ground reference map containing nine land-cover classes. (c) Original K-means clustering result (same-colored region belongs to the same subregion). (d) The result of image segmentation using the optimal K-means clustering method (each closed region is a subregion). The results of the ML classification after using different dimensionality reduction methods (number of features = 8), (e) OMNF method, (f) KMNF method, (g) OKMNF method, and (h) KM-KMNF method.
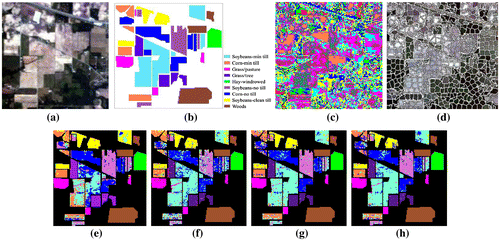
Table 3. Samples from Indian Pines used for training and testing.
Table 4. Overall accuracies of the ML classification on Indian Pines image using different dimensionality reduction methods.
Table 5. Overall accuracies of the SVM classification on Indian Pines image using different dimensionality reduction methods.
Figure 5. Comparison of the accuracies of the SVM classification on Indian Pines image after using different dimensionality reduction methods.
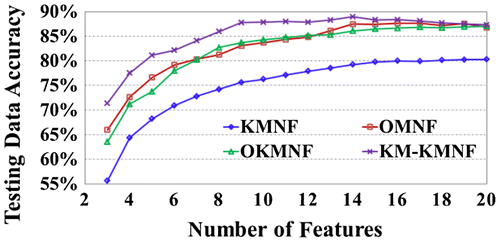