Figures & data
Figure 3. Line matching example: Real image (A) and corresponding synthetic image (B), each with 2 randomly sampled horizontal lines, sorted top to bottom (labeled as 1 and 2) and 2 randomly sampled vertical lines, sorted left to right (labeled 3 and 4). The real image lines’ corresponding virtual image line start and end points estimated by the point-to-line space resection (C).
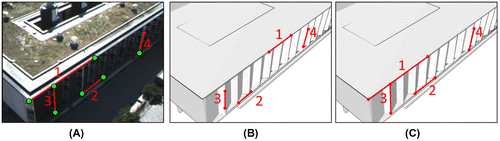