Figures & data
Figure 1. The architecture of the proposed network contains the backbone network, the coarse detection head and the Refining detection head, where the Backbone network extracts pyramid features of input images with feature pyramid network (FPN) (Lin et al. Citation2017a), the coarse detection head obtains a set of refined anchors and the refining detection head aims to achieve accurate object detection result. The regression loss used in the proposed network is IOU balanced loss
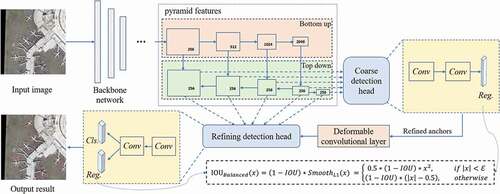
Figure 3. The schematic diagram of arbitrary orientated bounding box regression using smooth L1 loss. The axis and the
axis point to the positive direction of the screen coordinates. The term
denotes the angle from the positive direction of
axis to the direction of the long side of the predicted box where
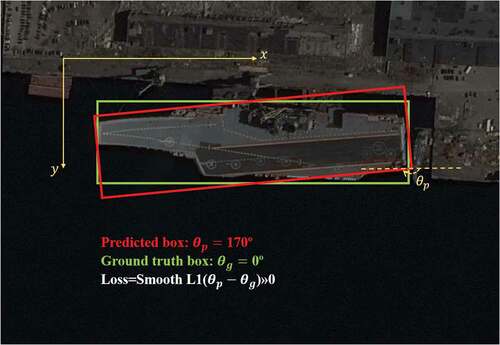
Table 1. Dataset description
Table 2. Detection result on DOTA.(%)
Table 3. Detection result on HRSC2016 (%)
Table 4. Speed-accuracy trade-off comparison with selected methods on DOTA dataset
Figure 6. Comparison of detection results on the HRSC2016 dataset with IOU balanced loss (even rows) and with smooth L1 loss (odd rows)
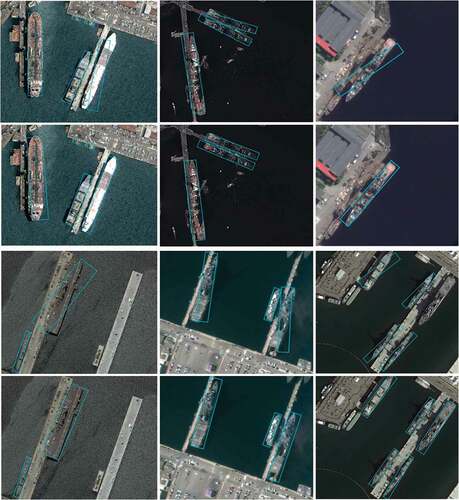
Table 5. Ablation study on HRSC2016 dataset
Data availability statement
The DOTA dataset that support the findings of this study is openly available in https://captain-whu.github.io/DOTA/dataset.html, and the HRSC2016 dataset that support the findings of this study is available from the corresponding author, upon reasonable request.