Figures & data
Figure 2. MODIS predictor variables for 01/01/2019: Terra Band 32 Emissivity (TE32, unitless, with a 0.002 scale factor), Terra Nighttime Land Surface Temperature (TLSTN, in kelvin with a scale factor of 0.02), Terra local time of night observation (TNVT, in hours with a scale factor of 0.1), Aqua Band 31 Emissivity (AE31, unitless, with a scale factor of 0.002), Aqua Daytime Land Surface Temperature (ALSTD, in kelvin with a scale factor of 0.02) and Aqua Nighttime Land Surface Temperature (ALSTN, in kelvin with a scale factor of 0.02).
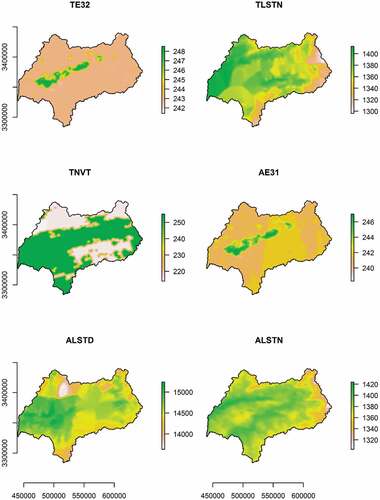
Table 1. Statistical and Machine learning methods used for Tair prediction
Table 2. Selected variables for PLS, RF, and XGB using forward feature selection
Figure 5. Agreement between measured air temperature and predicted air temperature using RF model as modeling tool. Models were first trained using the full set of predictor variables (ALL) and second using only selected variables as predictors that were revealed during forward feature selection (FS). Two different validation strategies were used: Comparison to a 70% random subset of the total dataset (RS), as well as Leave-One -Out Cross-Validation (LCV).
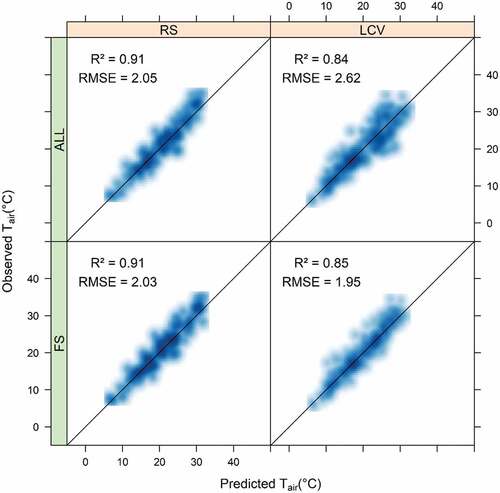
Figure 6. Correlation between measured and predicted air temperature based on KNN, PLS, XGB, XGBD, LM1, LM2, CUBIST, and RF models.
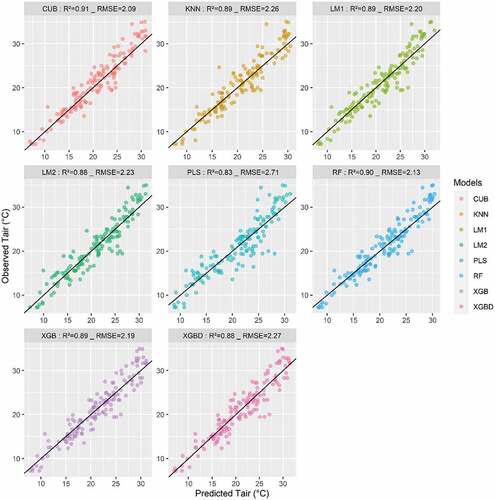
Figure 7. Distribution of residuals versus fitted air temperature based on KNN, PLS, XGB, XGBD, LM1, LM2, CUBIST, and RF models.
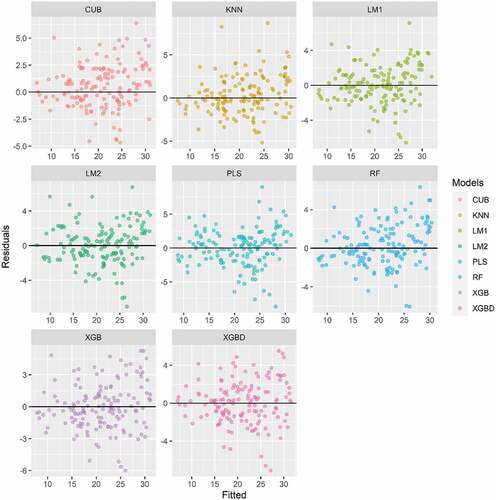
Figure 8. Time series for weather stations: Agadir Al Massira, Essaouira, Marrakech, and Ouarzazate weather stations for 2010–2019 period.
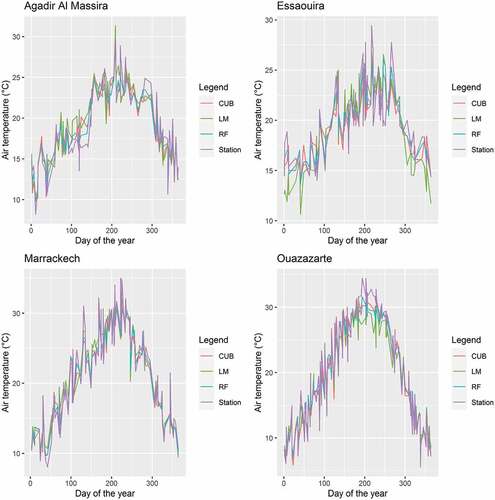
Figure 9. Station dependent errors: (a) RMSE for RF model, (b) Rsq for RF model, (c) RMSE for CUBIST model, (d) Rsq for CUBIST model, (e) RMSE for KNN model, (f) Rsq for KNN model, (g) RMSE for LM1, (h) Rsq for LM1, (i) RMSE for LM2, (j) Rsq for LM2, (k) RMSE for PLS model, (l) Rsq for PLS model, (m) RMSE for XGB model, (n) Rsq for XGB model, (o) RMSE for XGBD model, (p) Rsq for XGBD model. (For the stations: 1 = Agadir Al Massira, 2 = Essaouira, 3 = Marrackech and 4 = Ouarzazate.).
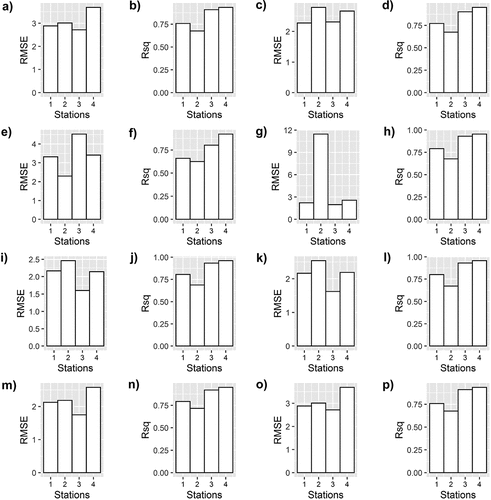
Data availability statement
The data that support the findings of this study are openly available at https://earthexplorer.usgs.gov/