Figures & data
Table 1. SDG 1 China’s district and county localization indicator system.
Table 2. Localized SDG 1 index weight for districts and counties.
Figure 4. SDG 1 evaluation value ranking of some districts and counties in China in 2014. (a) and (b) are calculated based on SDG 1 China’s district and county localization indicator system proposed in this study and by Wang et al. (Citation2021a) research, respectively. If the rank of the district/county is lower, it means that the SDG 1 evaluation value of the district/county is lower.
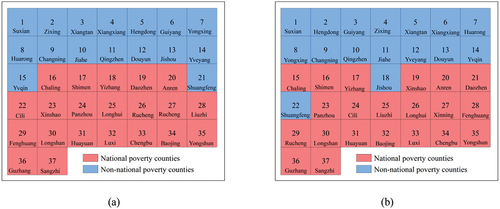
Table 3. Multidimension feature factors based on geographic data.
Table 4. Important parameters of the machine learning model.
Table 5. Results of SDG 1 China’s district and county localization estimation model.
Figure 6. The spatial distribution of NPCs and estimated NPCs of different models for China in 2014. (a) Distribution of NPCs. (b-f) Capacity of Model II, Model III, Model IV, Model V and Model VI to identify NPCs, respectively.
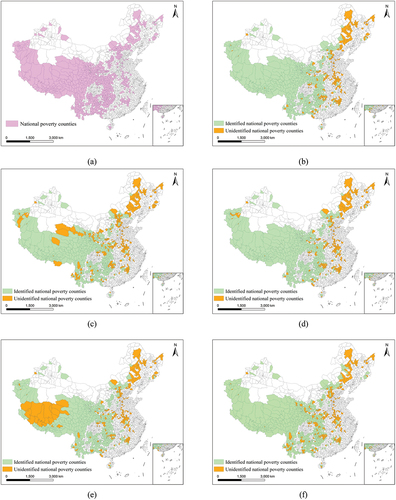
Figure 7. The spatial distributions of SDG 1 assessment values of China’s districts and counties in 2012, 2014, 2016, and 2018, respectively.
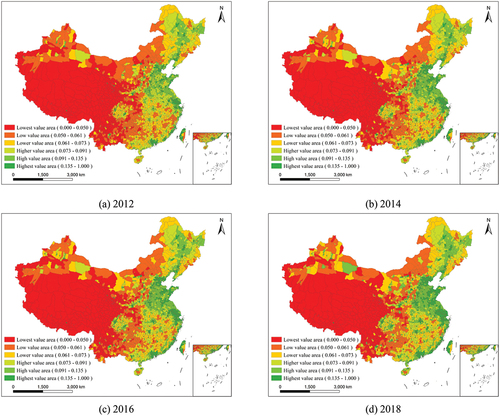
Figure 8. The changes in the SDG 1 assessment value of China’s districts and counties in 2012–2014, 2014–2016, 2016–2018, and 2012–2018, respectively.
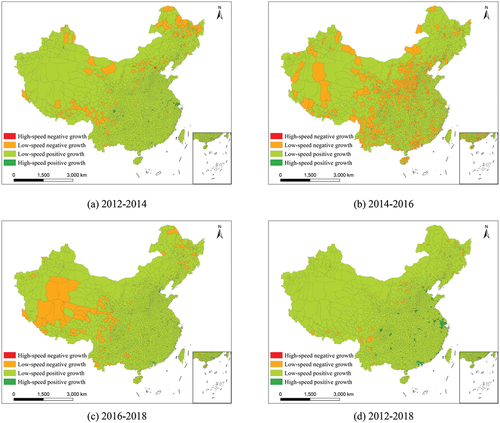
Figure 9. The local autocorrelation results of the SDG 1 evaluation value of China’s districts and counties in 2012, 2014, 2016, and 2018, respectively.
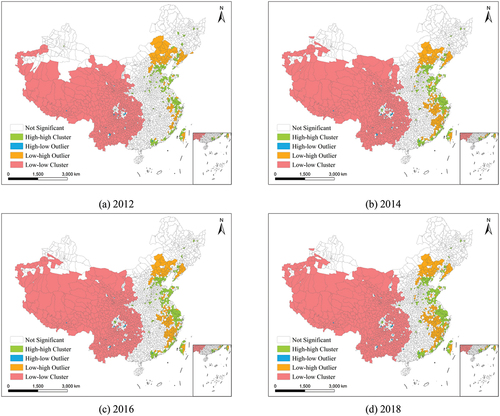
Figure 10. The standard deviation ellipse spatial distributions of the six graded areas of the SDG 1 evaluation value of China’s districts and counties in 2012, 2014, 2016, and 2018, respectively.
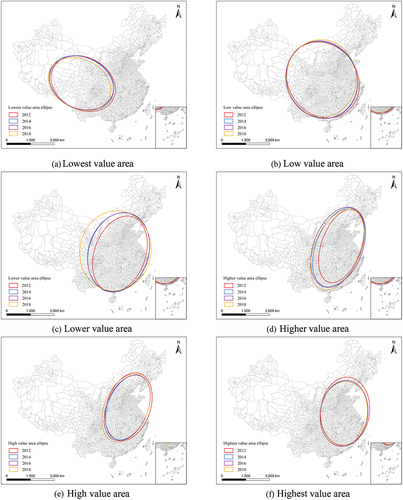
Figure 11. The track change of the ellipse’s center of gravity of the six graded areas of the SDG 1 evaluation value of China’s districts and counties.
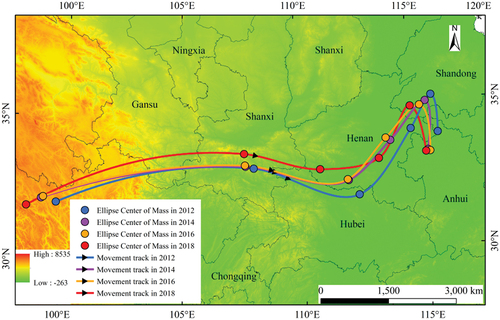
Figure 13. The spatial distribution of China’s SDG poverty counties’ estimated results in 2012, 2014, 2016, and 2018, respectively.
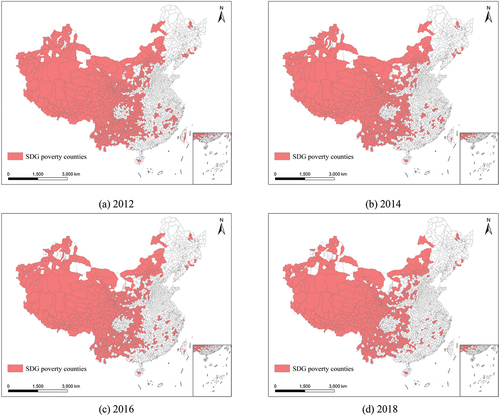
Table 6. Estimated results of related poverty monitoring studies.
Data availability statement
The data that support the findings of this study are available in the public domain:
SRTM DEM data: (https://doi.org/10.1080/10095020.2022.2108346)
NPP-VIIRS-like nighttime light data: (https://doi.org/10.1080/10095020.2022.2108346)
Land cover data: (https://doi.org/10.1080/10095020.2022.2108346)
Socio-economic statistics data: (https://doi.org/10.1080/10095020.2022.2108346)
Consent for publication
All the coauthors consent the publication of this work.