Figures & data
Table 1. Land-use classification results on GID with HRNetV2 model.
Table 2. Land-use classification results on GID with FCN8s model.
Figure 17. Process of adaptive optimization method for optimizing multi-scale and multi-channel image features.
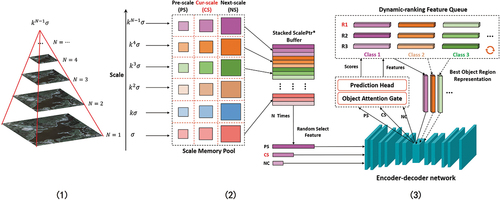
Table 3. Land-use classification results on WHU-Hi with FreeNet model.
Table 4. Scene classification results on WHU-RS19 with different parallel computing strategies.
Figure 26. Visualization on DOTAV2.0 (Ding et al. Citation2021) using Mask-RCNN (He et al. Citation2017) in object detection task.
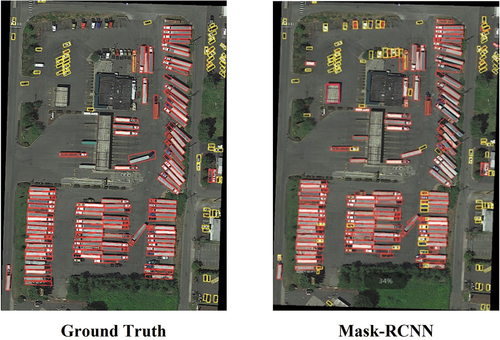
Figure 27. Visualization on ISPRS-Vaihingen (Rottensteiner et al. Citation2012) using U-Net (Ronneberger, Fischer, and Brox Citation2015) and DeepLabV3+ (Chen et al. Citation2018) in land-use classification task.

Figure 28. Visualization on WHU-BCD using DTCDSCN (Liu et al. Citation2020) in change detection task.
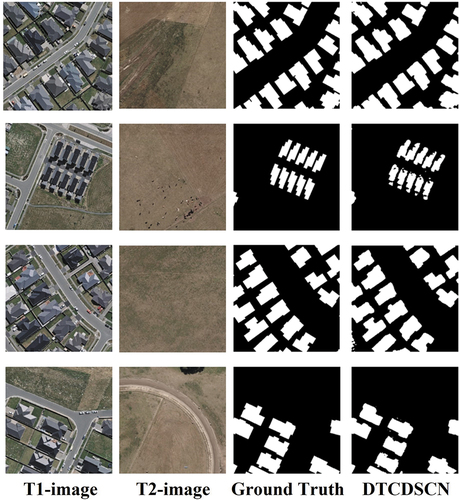
Figure 29. Visualization on WHU-Stereo (Li et al. Citation2022) using GC-Net (Ni et al. Citation2020) in multi-view 3D reconstruction task.

Data availability statement
The data that support the findings of this study are available on GitHub at https://github.com/WHULuoJiaTeam.